Introduction
The main priorities of e-Government initiatives are reducing administrative burdens; cutting costs; spurring innovation, and improving effectiveness and responsiveness (OECD, 2011). The implementation of the e-Government strategies generally follows very different routes with the implementation of Enterprise Architecture (EA) as one of the key initiatives. Enterprise Architecture provides a blueprint of the organisation’s information systems and facilitates continuous alignment of information and communication technology (ICT) to business strategy. Continuous innovation of Enterprise Architecture allows organisations to optimise the use of their ICT resources. Enterprise Architecture practice facilitates enterprise analysis and holistic design of information systems. Enterprise Architecture comprises of frameworks and methodologies that guide the transformation from the as-is situation of the enterprise to the desired future state (Mohamed, Galal-Edeen, Hassan, & Hasanien, 2012).
A framework is a logical arrangement for categorising and organising multiple and related information base of an entity (Chief Information Officer Council, 2001). EA frameworks (EAFs) provide an extensible skeleton for initial analysis and design of EA, and they are best suited to develop a vertical EA specific to a particular business domain (Sanchez, Basanya, Janowski, & Ojo, 2007). The four most commonly adopted frameworks as per the industry survey are Zachman Framework for EA (ZFEA), the Federal Enterprise Architecture Framework (FEAF), The Open Group Architecture Framework (TOGAF), and the US Treasury Enterprise Architecture Framework (TEAF) (Cameron & McMillan, 2013; Schekkerman, 2005; Session, 2007).
The four common frameworks have different objectives and attributes (Session, 2007). Their comparison is complex and poses severe challenges to the implementers of e-Government. The result of the framework selection commits the implementing government to a costly long-term undertaking, and if not done correctly, it will increase the risk of project failure. Problem-solving approach and levels of details differentiate Enterprise Architecture frameworks from each other. Most structures propose guidelines as the main feature, and others follow a methodological approach (Session, 2007). Most EAFs offer general and abstract approach to solutions making their validations and selection difficult.
Decision-making process at most public organisations employs experience, hunch, and lack of documentation instead of following proven scholarly prescriptions. The practice does not support reconcilable and useful quality data, especially within the public sector (Marcelo, Mandri-Perrott, House, & Schwartz, 2015). This weakness is also evident in choosing the critical component of e-Government solutions such as Enterprise Architecture framework. From the literature reviewed, there appears not a single process that is readily available that could be applied by developing countries to help them select an appropriate EAF (Bhupesh, 2015). The existing research shows that ranking and comparing enterprise architecture frameworks has been done for many purposes other than for e-Government solutions in developing countries (Bonnet, 2009; Cameron & McMillan, 2013; Mohamed et al., 2012; Session, 2007).
The research aims are to identify a systematic method to select EA framework attributes for an e-Government project. The research chose Botswana’s e-Government initiative as a case study due to its accessibility to the researchers. The research incorporated a qualitative approach based on the views from unstructured interviews with professionals and relevant discussions from workshops.
Background
As filtered from different literature sources, various methods are applied to analyse and compare existing Enterprise Architecture Frameworks to produce best of breed solutions. Mohamed et al. (2012) presented a comparative assessment of EAFs for e-Government. The research compares ZEAF, FEAF, TOGAF and TEAF. A criterion for the appraisal of enterprise architecture for e-Government by analysing the challenges of e-Government development was adopted. The research categorised the evaluation along with quality requirements and development issues, including Enterprise Architecture support standardisation and collaboration between government agencies. The study conducted by Mohamed et al. (2012) outlines the strengths and weaknesses of different frameworks and gives the reader an insight that some of the frames, such as FEAF and TOGAF have convergent results but does not provide a clear guide of how frameworks can be blended.
Session (2007) compares different sets of Enterprise Architecture Methodologies. He analyses the ZEAF, Gartner Enterprise Architecture Framework (GEAF), and TOGAF. Session (2007) uses a qualitative approach to framework evaluations by first categorising the frameworks as either framework in the real sense or methodologies. He concluded that ZEAF is a taxonomy and not a framework. He argued that ZEAF is a taxonomy for organising architectural artefacts. ZEAF prescribes view perspective and target artefacts as opposed to setting and detailing abstract concepts and values.
Session (2007) argued further that TOGAF is a methodology and not a framework. He considers FEAF similar to Zachman as they are both comprehensive categorisation of Enterprise Architecture artefacts. He considers FEAF an architecture development process comparable to TOGAF as they both deploy an architecture development methodology (ADM). He argues that FEAF is not a framework but a development methodology for building enterprise architecture. It is the result of applying the ADM to the U.S. Government. (Session, 2007) concludes that Enterprise Architecture frameworks are all different, and therefore, the process of selecting a framework is a multi-criterion problem.
Mohamed et al. (2012) highlight the fundamental differences of the compared architecture methodologies. He uses a set of criteria for a fictional company (enterprise) and awards ratings for each method. The research concludes that the approaches are complementary, and the best solution for many companies is having all the methodologies intermingled according to the way that suits the organisation’s constraints, but the study appears to lack the qualitative approach that informs the extent of the blended solution. Urbaczewski and Mrdali (2006) compare several Enterprise Architecture Framework that meets the needed criteria. They compared ZFEA, DoDAF, FEAF, TEAF, and TOGAF. Their method of comparison established a precise definition of an enterprise architecture framework.
Due to the challenges in comparing the EAF, Urbaczewski, and Mrdali (2006) chose to relate the five EAF according to the EAF views, level of abstractions, and how they facilitate the Systems Development Life Cycle (SDLC). The research establishes that the Zachman framework exhibit more characteristics of a typical framework than others do as it uses several viewpoints concerning different aspects. They point out that a challenge exists when utilising the perspectives as some frameworks fail to adequately translate “viewpoints” as “aspects” such as in the rows and columns as described in the ZEAF. They recommended further research to quantify the process to select Enterprise Architecture frameworks that align with the specific objectives of an organisation.
Ssebuggwawo, Hoppenbrouwers, and Proper (2010) investigate the concept of architecture by scrutinising six architecture frameworks (AFs). The purpose of their research was to analyse and compare framework similarities and differences. Ssebuggwawo et al. (2010) defined goal, inputs and outcomes as essential elements that could characterise AFs. They analyse different standpoints or perspectives used to model architecture. The research did not cover the presentation or representation used by the EAFs. They found that some EAFs were non-specific on the depictions of their views, but other frameworks suggested the use of standardised descriptive languages. Their recommendation, therefore, is that there is a need to implement a research instrument that will track the interactions of modeller’s preferences and their priorities.
In a more analytical examination of Enterprise Architecture Frameworks; Magoulas, Hadzic, Saarikko, and K. Pessi (2012) focus on explaining “How are the various forms and aspects of architectural alignment treated by the investigated approaches to Enterprise Architecture?”. The research proposes an analysis and an alignment of various forms and aspects of structural arrangement which were treated by formalised approaches to EA. Magoulas et al. (2012) utilise the Massachusetts Institute of Technology (MIT1990s) framework for organisational research as a baseline for evaluating Enterprise Architecture frameworks. The recommendation, therefore, is that corporate powers rather than rational thinking are responsible for architectural patterns. The general concept makes the Enterprise Architecture framework selection a much more complicated task even though the selection is usually trivialised and left only to the consulting companies with little input from the management.
The authors observe the many different subjective arguments coming from the literature, and there is no definite recommendation for the ‘best-fitting’’ method. The problem is complex, with various competing alternatives and the criteria used for comparison. Hence, this research recognises a need to deploy quantitative methods that implore measuring factors that could influence a good architecture. Such factors could then be used to compare the different architectures in the form of a decision problem. Since there are many factors and some alternatives, the process is termed a multicriteria decision making (MCDM) problem and requires an MCDM analysis, that is, the application of the AHP method.
Application of Multiple Attribute Decision Analysis
Multiple-criteria decision analysis (MCDA) is a decision-making disciple that systematically takes into consideration different characteristics of an entity. MCDA methods utilise a set of a decision matrix to systematically analyse an approach for mitigating risk levels, vagueness, and assessment, which enables the decision making and classification of many alternatives according to preferences ratings (Linkov & Steevens, 2008). MCDA facilitates decision-making processes by standardising the planning and structuring of the decisions problem. The objective is to provide the decision-maker with an objective and balanced decision taking into consideration the weights and importance of alternatives. Lack of simplicity due to the existence of choices in MCDA creates decision-making problems. In a typical MCDA problem, there is no clear optimal solution that exists. It then becomes necessary to aggregate decisions of more than one decision-maker through a systematic process. A set of non-dominated solutions replace the concept of the best solution (Linkov & Steevens, 2008). In identifying the non-dominated solutions, different techniques are used to differentiate between acceptable and unacceptable alternatives (Linkov & Steevens, 2008). Magoulas et al. (2012) provided a model to analyse complex problems and assist decision making as to:
- Describe and denote problem attributes explicitly
- Provide an instrument for assessing the level of attribute realisation in diverse situations.
The setting requires a multiple-criteria decision-making (MCDM) approach where multiple criteria and alternatives exist, and a decision-maker(s)’s task is to select the most appropriate decision given their preferences. From the literature on MCDM techniques, Analytical Hierarchy Process is one of the most widely and accepted methods. Analytical Hierarchy Process (AHP) can be used for ordering and choosing the preferred candidates from amongst contenders (Saaty, 2008) To prioritise; decision-makers can apply different methods such as subjective judgement with or without consensus-building. Most techniques do not provide a measure of the goodness of the decision ranking. To address this weakness, AHP provides consistency index for grading. The prioritised options use pair-wise comparisons. An advantage of AHP is its ability to handle quantitative analysis, and its results can be validated (Liimatainen, Hoffmann, & Heikkilä, 2007). AHP is simple, applicable in many situations and can mix measurable and non-measurable analysis. However, AHP is unable to solve problems when decision-making cannot make clear cut decisions. Introducing fuzzy AHP can improve the base AHP where exact (or crisp) numbers represent human’s judgements even though this is not the case in reality (Davoudi & Sheykhvand, 2012; Gardasevic-Filipovic & Saletic, 2010; Sehra, Brar, & Kaur, 2012).
The research, therefore, explored to use AHP, as it is easy, flexible, and intuitive. It provides the capability to blend numerical and non-numerical criteria in the same decision framework. AHP is also highly recommended and appropriate for collaborative decision making (Ssebuggwawo et al., 2010).
Research Methodology
The research identifies several possible candidates for addressing the multi-criteria analysis problem. The first task to compare the Enterprise Architecture frameworks using AHP is to set the goal. The goal is to select one or more frameworks that best fit the defined requirements for e-Government implementation.
A criterion set to enable the evaluation of the Enterprise Architecture frameworks was defined based on the critical success factors identified from the interviews with e-Government officials and literature reviews (Mokone, Eyitayo, & Masizana-Katongo, 2018). Table 1 shows the criteria (Ci) and sub-criteria (Ci.j):
Table 1: Criteria and sub-criteria
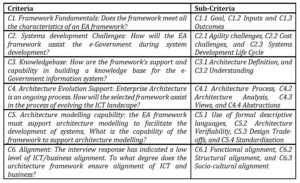
Alternatives
The four common enterprise architecture frameworks for e-Government, as identified in the literature review are:
Alternative 1 (A1): ZEAF
Alternative 2 (A2): FEAF
Alternative 3 (A3): TOGAF
Alternative 4 (A4): TEAF
EA framework selection using AHP
Using the AHP decomposition steps (Adamcsek, 2008; Srdevic, Blagojevic, & Srdevic, 2011; Ssebuggwawo et al., 2010), the research decomposed the EA framework selection needed to achieve the set goal as shown in Figure 1.
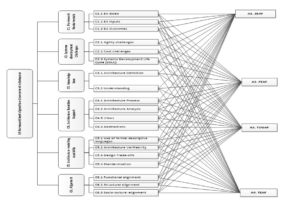
Figure 1: AHP Hierarchy
The AHP hierarchy shows the Goal (g), main criteria (c), sub-criteria (ci.j) and alternatives (a). AHP specifies the goal at the top level, followed by rules and the other options. It then displays the options at the lowest level. The sub-criteria displayed in the middle of the hierarchy refines the requirements.
AHP Solution to select EA framework
The research developed an AHP system to select the most suitable framework for e-Government in Botswana. The system design followed the AHP hierarchy identified in Figure 1.
Main Criteria and Sub criteria Priorities
The AHP algorithm converted selections into AHP matrices to calculate the priorities of each selection preference. Arithmetic mean method underpins the resultant priorities. The simplicity and intuitiveness of the arithmetic mean method made it a better choice over the eigenvector method and the geometric mean. Wu, Chiang, and Lin (2008) stated that there is no difference among the three methods until the number of experts exceeds two hundred and the criteria are less than four, and when experts exceed three hundred and the criteria is less than five. The current research used less than fifty experts and nineteen criteria.
Local Matrix and attribute priorities for Sub-Criteria
For each alternative in Figure 1: AHP Hierarchy, local priorities were calculated for each sub-criteria using the arithmetic mean method. Example 1 shows the steps to calculate and populate the local decision matrix for C1.1 EA Goal.
Example 1: Decision matrix and local priorities for Frameworks given C1.1 EA Goals:
Given ZEAF in Figure 1: AHP Hierarchy, the following steps show the process that was followed by the decision-makers and which were required to rate their preference of ZEAF given other frameworks:
Step 1: Derive decision maker’s preference matrix
using Saaty’s ration scale; the research made a comparison amongst alternatives at the same level given a particular attribute. The first comparison compared the importance of a framework over another assigned EA Goals and scores captured in Table 2. To achieve the results, ask the following question repeatedly – Given EA Goals, which framework is preferred and by how much? Then reiterate the process for the top half of the matrix until completing all comparisons. To finish the lower part of the matrix, invert the high scores using the reciprocity principle.
Step 2: Calculate sum of the column
to calculate the sum of the columns, add all matrix elements under the same alternative. For example, the following column sum was derived given ZEAF:
ZEAF = 1 + 0.5 + 0.25 + 0.11 = 1.86
Repeat the process for all alternatives and then derive the sums as follows;
FEAF = (2 + 1 + 0.2 + 0.25) = 3.45
TOGAF = (4 + 5 + 1 + 0.25) = 10.25
TEAF = (9 + 4 +4 + 1) = 18
The comparisons and their additions were then populated into the first part of Table 2 to complete the decision matrix.
Step 3: Derive weight of the alternatives matrix
In order to derive the weight of the alternative matrix, divide each entry of the decision matrix by the sum of the preference value. To calculate the preference weight of ZEAF to ZEAF, perform the following calculations;
Preference weight for ZEAF to ZEAF = Preference Value/sum of preference values
= 1/1.86 = 0.537
Repeat for all the entries given ZEAF as follows;
Preference weight for ZEAF to FEAF = Preference Value/sum of preference values
= 2/3.45 = 0.579
Preference weight for ZEAF to TOGAF = Preference Value/sum of preference values
= 4/10.25 = 0.390
Preference weight for ZEAF to TEAF = Preference Value/sum of preference values
= 9/18 = 0.5
To complete populating the weight of alternative matrix in the lower part of Table 2, repeat the process for all entries of the decision matrix.
Step 4: Calculate the local priorities
To calculate the local priorities, calculate the average value of the entries from the weights of the alternatives. For example, to derive the local priority for ZEAF, the following average was calculated;
Local Priority for ZEAF = (0.537 + 0.579 + 0.390 + 0.5)/4 = 0.501
Repeat for all alternatives to complete Table 2.
Table 2: Local Priorities for Frameworks given C1.1 EA Goals
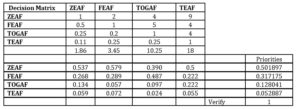
Follow Steps 1 – 4 above to derive decision matrix and local priorities for all sub-criteria C1.2 to C1.3.
Step 6: Compile local priorities
From the calculated preferences, derive Matrix_Cn for each set of sub-criteria. Table 3 shows the matrix derived by compiling the local preferences of C1.
Table 3: Local Matrix_C1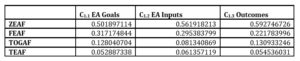
To derive the rest of the matrix for other alternatives from Figure 1: AHP Hierarchy, follow Steps 1 – 6 for all criteria C2.1 to C2.3, C3.1 to C3.2, C4.1 to C4.4, C5.1 to C5.4 and C6.1 to C6.3.
Local Priorities and Preference Vectors for main Criteria, Cn
This section derives local priorities and preference vectors for the main criteria, Cn from Figure 1: AHP Hierarchy. To each priority, follow Steps 1 – 6 from Section 4.3.2. For example, to calculate the local priority for EA Goals, perform the following calculations;
EA Goals = Sum of Preference Values compared to EA Goals
= (Goals Vs Goals) + (Goals vs Inputs) + (Goals vs Outcomes)
= 1 + 1.19 + 1.18
= 3.37
EA Inputs = Sum of Preference Values compared to EA Inputs
= 2.27
EA Outcomes = Sum of Preference Values compared to EA Outcomes
= 4.18
To determine the local weight, divide each preference value in the decision matrix by the sum of the local preference values;
For EA Goals, Local Weight (1, 1) = (1/3.37) = 0.296
Local Weight (1, 2) = 0.84/2.27 = 0.370
Local Weight (1, 3) = 0.85/4.18 = 0.203
To calculate the Local Priority;
Local Priority for EA Goals = (0.296 + 0.370 + 0.203)/3 = 0.290
The preference vectors were identified from the priorities of each attribute as shown in Figure 2.
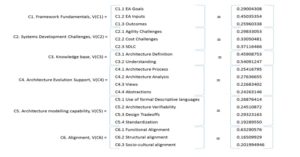
Figure 2: Preference vectors, V (Cn)
Aggregation Matrix
An aggregate matrix calculated from the alternatives selects the preferred EA framework through decision matrices and the preference vector, as shown in Table 4. Using the matrix algebra, a four by three [4 x 3] matrix multiplied by a three by one [3 x 1] matrix gives a four by one [4 x 1] matrix. The output provides the first entry, ZEAF (Ci) as follows where ZEAF (C1) is from Table 2;
ZEAF (C1) = (0.501*0.29) + (0.561*0.450) + (0.593*0.259) = 0.552
Calculate the local value of all frameworks given C1. Framework Fundamentals as follows;
= Matrix(C1 ) x Vector(V (C1)) where
C1 = Table 3: Local Matrix_C1
and,
Vector, V (C1) = C1.Framework Fundamentals,
V(C1) = [C1.1 EAGoals],[C1.2 EA Inputs], [C1.3 Outcomes]
= [0.29004308],[0.45035354],[0.25960338]
Table 4: EA combination matrix
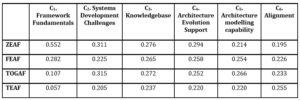
To complete the EA framework ranking, add local priorities and divide by the number of entries. For an example, the aggregate rating for ZEAF was calculated as follows;
ZEAF = (0.552 + 0.311 + 0.276 + 0.294 + 0.214 + 0.195) / 6
ZEAF = 0.307
Perform the calculations for all frameworks and the results in a table, Table 5. In this research, ZEAF ranked first, FEAF second, TOGAF third and TEAF last. The rankings of the frameworks show an order of preference for the framework attributes when selecting an EA framework for e-Government in Botswana.
Table 5: EA Framework ranking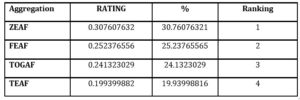
Discussion
The authors developed a process using AHP to rank the most common Enterprise Architecture frameworks used in e-Government. AHP provides an advantage of allowing comparisons of the attributes of the frameworks. The results rank ZEAF first at 30.7%, FEAF second at 25.2%, TOGAF third at 24.1% and TEAF fourth by 19.9%. When using a simple arithmetic summation to check the consistency of the values, the research found that the totals add to 100.07%. These results show an error of
+0.07%. However, using non-crisp values and group decision making can improve the results.
From the combination matrix, ZEAF outperformed all the other frameworks on the capability to support the Framework fundamentals, Enterprise Architecture knowledge base and architecture evolution support. TOGAF’s most preferred attributes are the ability to handle Systems Development Challenges and Architecture modelling capability. The research found that business alignment to ICT is the most favoured strength of TEAF. FEAF attributes did not appear to be the most preferred on an individual basis, but the framework was the second overall preferred, see Table 6.
Table 6: Framework attributes preference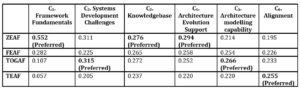
The results are consistent with the literature review. ZEAF define the primary taxonomy that facilitates the formulation of many Enterprise Architecture frameworks. Many of the current Enterprise Architecture frameworks used in e-Government projects were influenced heavily by ZEAF. On the other hand, FEAF was designed by the USA CIO council to meet the requirements of Governments. It, therefore, contains exhibits alignment to USA e-Government by design. However, as has been identified in the literature review, no EA framework fits all e-Government projects. TOGAF is rated fourth, which agrees with its position as the private sector leads open architecture framework based on TEAF, ZEAF and TAFIM.
Conclusion and Recommendations
The EA framework supports the implementing organisation to understand its ICT environment, to document all EA domains, to support structured EA artefact repositories, to support SDLC and to align ICT with business. However, existing EA framework selection methods are not adequate as they assume a high level of ICT and e-Government maturity.
This paper utilises a multi-criteria decision method to select a framework that meets the critical success factors of e-Government. The implementing organisation should first establish a goal for the success criteria using an appropriate MCDA method. The goal-setting forms the business demand side. The second phase involves subjecting available EA frameworks to the required attributes to determine the most appropriate EA framework. To conclude the alignment, the implementing organisation must take into considerations the enablers needed for successful implementation of e-Government. In this research, the Zachman Enterprise Architecture Framework ranked highest at 30.7% for implementing Enterprise Architecture in Botswana.
The research contributes to furthering the knowledge of Enterprise Architecture and provides a basis for further research within the Enterprise Architecture and e-Government fields. Developed countries have demonstrated that a well-deployed Enterprise Architecture can have a positive impact on the success of e-Government. Enterprise Architecture frameworks selection is a difficult task that requires consideration of many factors. This paper proposes an application of AHP to select an appropriate EA framework for e-Government to mitigate risks where there is low ICT maturity.
The paper contributes to reducing the gap in the understanding of Enterprise Architecture and the selection of enterprise architecture frameworks. The research community should conduct further research on the deployment of enterprise architecture frameworks for better results. There is also a need to carry out more investigations on the adoption of architecture for new technologies.
The research is consistent with the literature review and demonstrates value in adopting AHP for selecting frameworks. AHP algorithm provides an intuitive and quantitative method to choose an Enterprise Architecture framework.
Acknowledgement
The current research paper is the second part of the research work by Mr Mokone in partial fulfilment of his requirements for MSc in Computer Information Systems with the University of Botswana. The authors would like to thank the Ministry of Transport and Communications (MTC) and e-Government Offices in Botswana for allowing this research to be carried out. The first publication covered the identification of the Critical Success Factors (CSF) of e-Government for the case of Botswana with the same authors.
(adsbygoogle = window.adsbygoogle || []).push({});
References
- Adamcsek, E., (2008). The Analytic Hierarchy Process and its Generalizations. PhD Thesis, pg. 1-43.
- Bhupesh, M., (2015). Building IT Architecture for Developing nations Using TOGAF. Innovation and Software, Computer Science, 1-75.
- Bonnet, M. J. A. (2009). Measuring the Effectiveness of Enterprise Architecture Implementation. Faculty of Technology, Policy and Management Section Information & Communication Technology, Master of Science in Systems Engineering, Policy Analysis and Management – Information Architecture, pg. 1-90.
- Cameron, B. H., & McMillan, E. (2013). Analysing the Current Trends in Enterprise Architecture Frameworks. Journal of Enterprise Architecture, pg. 60-72.
- Chief Information Officer Council. (2001). A Practical Guide to Federal Enterprise Architecture. 112p
- Davoudi, M. R., & Sheykhvand, K. (2012). Enterprise Architecture Analysis Using AHP and Fuzzy AHP. International Journal of Computer Theory and Engineering, 4(6), pg. 911.
- Gardasevic-Filipovic, M., & Saletic, D. Z., (2010). MULTICRITERIA OPTIMIZATION IN A FUZZY ENVIRONMENT: THE FUZZY ANALYTIC HIERARCHY PROCESS. Yugoslav Journal of Operations Research, 20(1), pg. 83.
- Liimatainen, K., Hoffmann, M., & Heikkilä, J. (2007). Overview of Enterprise Architecture work in 15 countries. Finnish Enterprise Architecture Research Project. pg.1-81.
- Linkov, I., & Steevens, J. (2008). Chapter 35 Appendix A: Multi-Criteria Decision Analysis. Paper presented at the ADVANCES IN EXPERIMENTAL MEDICINE AND BIOLOGY; 619; 815-830.
- Magoulas, T., Hadzic, A., Saarikko, T., & K. Pessi. (2012). Alignment in Enterprise Architecture: A Comparative Analysis of Four Architectural Approaches. Electronic Journal of Information Systems Evaluation, 15(1), pg. 88-101.
- Marcelo, D., Mandri-Perrott, C., House, S., & Schwartz, J. (2015). Prioritization of Infrastructure Projects: A Decision Support Framework. 1-30. Retrieved from http://g20.org.tr/wp-content/uploads/2015/11/WBG-Working-Paper-on-Prioritization-of-Infrastructure-Projects.pdf.
- Mohamed, M. A., Galal-Edeen, G. H., Hassan, H. A., & Hasanien, E. E. (2012). An Evaluation of Enterprise Architecture Frameworks for E-Government. IEEE, pg. 255-261.
- Mokone, C. B., Eyitayo, O. T., & Masizana-Katongo, A. (2018). Critical Success Factors for e-Government Projects: The case of Botswana. Journal of E-Government Studies and Best Practices, 2018(2018), 1-14. Retrieved from https://ibimapublishing.org/articles/JEGSBP/2018/335906/. doi:10.5171/2018.335906
- (2011). E-government strategies. In Government at a Glance 2011 (pp. pg. 98-99): OECD Publishing.
- Saaty, T. L. (2008). Decision making with the analytic hierarchy process. J. Services Sciences, 1(1), pg. 83-99.
- Sanchez, A., Basanya, R., Janowski, T., & Ojo, A. (2007). Enterprise Architectures – Enabling Interoperability Between Organizations. 8th Argentinean Symposium on Software Engineering, part of 36th Argentine Conference on Informatics, ASSE 2007, pg. 1-10.
- Schekkerman, J., (2005). Trends in Enterprise Architecture 2005: How are Organizations Progressing? , pg. 1-33.
- Sehra, S. K., Brar, Y. S. & Kaur, N. (2012). Multi-Criteria Decision Making Approach for Selecting Effort Estimation Model. International Journal of Computer Applications, 39(1), pg. 10.
- Session, R. (2007). Comparison of the Top Four Enterprise Architecture Methodologies. In Microsoft (Ed.), (pp. pg. 1-46).
- Srdevic, Z., Blagojevic, B., & Srdevic, B. (2011). AHP based group decision making in ranking loan applicants for purchasing irrigation equipment: a case study. Bulgarian Journal of Agricultural Science, 17(4), pg. 531-544.
- Ssebuggwawo, D., Hoppenbrouwers, S., & Proper, H. (2010). Group Decision Making in Collaborative Modeling – Aggregating Individual Preferences with AHP. 5th SIKS/BENAIS Conference on Enterprise Information Systems, pg. 1-12.
- Urbaczewski, L., & Mrdali, S. (2006). A Comparison of Enterprise Architecture Frameworks. Issues in Information Systems, Volume VII(2), 3. Retrieved from http://iacis.org/iis/2006/Urbaczewki_Mrdalj.pdf.
- Wu, W.-H., Chiang, C.-t., & Lin, C.-t. (2008). Comparing the aggregation methods in the analytic hierarchy process when uniform distribution. WSEAS TRANSACTIONS on BUSINESS and ECONOMICS, 5(3), pg. 82 – 84.