Introduction
With the whole pandemic going on and the overall growth of e-learning and virtual assistants over the last years, online teaching aims to ensure the most effective and safe experience for its students. As higher education institutions rely more on digital platforms to build learning experiences, it is important to consider how the change in teaching practices increases student engagement. Individuals are most spurred when they realize how helpful the e-learning exercises are for their academic development. The positive attitudes towards learning are due to planning flexibility, lessened expenses and time saving, where students have the chance to learn paying little mind to area or time [5].
On this matter, the purpose of our study is to establish the basics for designing an interactive virtual “teacher” with the help of AI (artificial intelligence), to analyze the skills required by instructors to deal with undergraduates’ requirements in university courses, and consider their needs, level of commitment, and sense of identity with the proposed situation. With study/teaching platforms modified to a student’s necessities and inclinations, almost certainly, there will be an increase in their determination to learn, as it is more appealing to learn in a setting that is comfortable for them. Online courses can possibly altogether build the opportunities for inexpensively quick examination through access to truly extending information based on e-texts, lists of sources and connections to significant possessions in college libraries all throughout the planet.
Initial Evaluation – Survey
Taking a look at the survey held at Dhaka University, Bangladesh (Aug 2020) [8], which covered data from 150 students, it is noted that 94% of the students use the Internet and only 6% of them do not use it currently. From the total of 94%, 60% are seeking help from Google for academic purposes regularly. In addition, a vast majority of the students imply that the Internet is to thank for the great increase of their academic performances. Taking into account this information, it seems that undergraduates are increasingly less thrilled to flip through books or reach out to their teachers when it comes to school-related issues.
Now getting back to our original task, we need to prove the efficiency of a virtual instructor and have a better understanding of the students’ needs and preferences. In this regard, we conducted a small survey for: Faculty of Electronics, Telecommunications and Information Technology, University of Medicine and Pharmacy Carol Davila, Faculty of Veterinary Medicine & The Bucharest University of Economic Studies, which involved answers from a total of 300 students. Specifically, this study examined the following research questions:
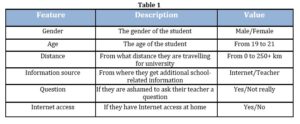
As shown below (Fig 1, Fig 2), the majority of them declared that sometimes they feel ashamed to ask their teacher certain questions, so they prefer to consult the Internet when encountering school-related issues:
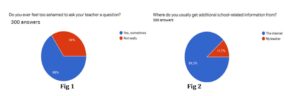
That’s hardly surprising, taking into account how much easier it is to spare yourself the fuss of deciding whether your question is ridiculous or not, then waiting for an answer after finally making the courage to ask, when you could simply type it on Google instead. With the improvement in Internet industry, i.e., web assets; searching for school materials has now turned into a question of a couple of snaps. Presently, undergraduates can get data on any point relating to science through web search tools. However, you can never guarantee that all websites are giving fully accurate information, since everyone is allowed to post on the Internet, even non-licensed authors. Therefore, since students rely so much on the Internet when gathering facts, why not creating a reliable, trustworthy platform where you can get your answers from, without worrying that the information might be wrong? There are already many successful apps for learning languages like Babbel or Duolingo with millions of active users, which proves that a virtual “teacher” can help you improve your skills or even become fluent from scratch. Now imagine there was an app which contained every field of study, from software engineering and designing to sociology and science, giving students the opportunity to express themselves openly through avatars. The entire motivation behind having unknown characters is to empower learners to face challenges with their intuition, to create concepts, and tackle issues.
In many cases, students feel uncomfortable when they have to seek help from other people. When direct human engagement is problematic and teachers believe it menaces their authority or dignity, virtual mentors can clearly be beneficial. A robot [1] is never too busy for you, it’s also not capable of judging any curiosity you might have, even when dealing with personal oddities (e.g., body-related issues that you don’t feel comfortable discussing with your biology teacher; physics or grammar doubts that may seem too silly to ask about).
Virtual teachers may respond and adjust to each learner, customizing education to specific requirements, unlike a passive textbook. There is strong evidence that they can potentially help children acquire important knowledge, such as teaching them about their medical issues, developing and practicing learning, and then assessing it. Being just a digital work, they can also undertake teaching responsibilities that would be time-consuming, unpleasant, constraining, or impossible for human teachers to do. They can, for example, patiently assist a student in practicing a skill or technique, practicing a foreign language conversation, or act silly and pretend to need help from the student so that it stimulates their oratory skills.
The next question (Fig 3) concerned the distance that students learning in higher education need to travel for pursuing their studies. As it turns out, 57% of them have to attend courses from over 100km away (which is already pretty far), with 16% travelling a distance even longer than 250km.
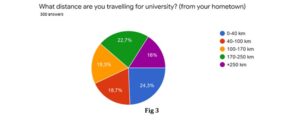
With ViTeach, however, their classroom would only be one click away. Students can pursue academics while working or maintaining their own schedules through distance learning. Having access to all of the resources of a standard course allows members to learn in any place, giving them the opportunity to study whenever they want [7]. Any student can thus attend all kinds of courses using only an Internet connection. The expenditures and time wasted to make it to college could be invested in studying from their home through the virtual learning environment. Besides giving an elective method to learn in the advanced age, online courses offer grown-ups and working experts the chance to acquire new abilities or improve existing ones. The benefit is that they can login to the framework whenever and begin following the module. They can also engage in conversations with their fellow students through the chat provided, join study groups or a reading club; or interact with the staff by using the suggestion box.
The course content in distance learning can be delivered via audiotapes, recordings, the Internet, or a printed structure. Distance learning, with the use of current technology, has a more extensive variety of techniques of correspondence than traditional study hall learning. Henceforth, distance learning will no longer be lacking in correspondence. Undergraduates enrolled in distance learning programs do, in fact, have more correspondence options.
Designing The Environment
For a better representation of this whole concept, we need to provide a visual attribute by showing an example of how the platform could look like. As shown below, the app has a general menu with 5 options that students have to choose from, including a chat (Fig 14), a suggestion box (Fig 13) and a new feature called “Do not disturb” (Fig 6).
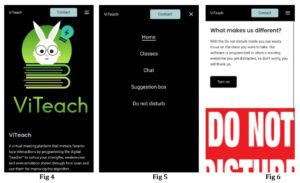
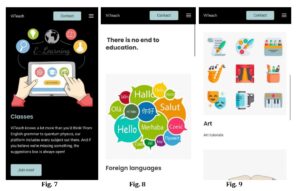
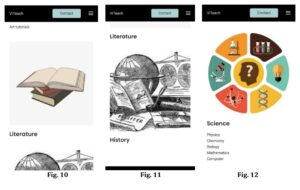
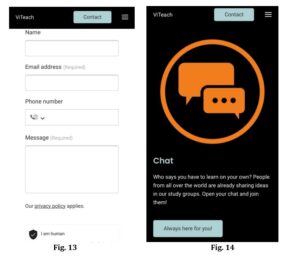
The first two options (Fig 5) called “home” (Fig 4) and “classes” (Fig 7) mostly speak for themselves, involving just the main page and the school subjects that students want to take. The chat, on the other hand, is a little more unusual, offering a wide range of discussions that students can browse through and join, or even create their own. In the case of opinion groups, they pick a side – pro or con – and share their own beliefs. Since interaction is essential in a learning environment, we promote a different approach by creating a diverse network, rather than a generic, task-centralized one. By connecting a large number of individuals through various groups of interest, not only that the volume of transferred information is increased, but the productivity is boosted. This is the reason why the undergraduate can join as many group chats as he wants, as it can enhance team spirit and promote individual growth [6]. In a web asynchronous conversation, the individual may reconsider his response or take a moment to consider others’ opinions before speaking or skipping to the following item. This structure gives them more time and space to compose answers with rather more deliberation and precaution than in a conventional eye-to-eye conversation, where the speakers have to analyze each other’s comments on the spot and prepare a response or lose the opportunity to participate in the discussion. Furthermore, the communication channels allow the students to ask as many questions related to the course or their assignments at any time, without worrying about the inadequate hour.
The next option, named “suggestion box” (Fig 13), is actually what keeps the algorithm alive; the public opinion matters the most when creating a platform dedicated to people. Here, learners have the possibility of sharing their ideas with us and improving the virtual “teacher” to match their needs and preferences. When students are hidden behind a computer, it is easier for them to share dissenting ideas or “outside-the-box” ideas. Generally speaking, the reasoning behind such architecture is the need to enhance the experience of the learner, and we believe that this can be acquired through a process of continuous interaction, often overlooked in common environments.
The battle with focusing on the screen for exte1nded periods of time is probably the most difficult test of Internet learning for some students. With online learning, there is also a greater possibility for undergraduates to be immediately diverted off track by social media or other apps. This is why it feels necessary to have a “do not disturb” option as well, for the learners who want to be monitored during the ongoing classes so that they won’t get distracted and focus on their studies instead. During the study hours, the AI (artificial intelligence) computes muscle points on the learners’ faces through the front camera on their phone or computer, and thus recognizes feelings such as confusion, happiness, anger. To give a more detailed description of this feature, we’ll explain the process in a relatively technical manner: The front facing camera of the student’s device perceives the key points marked to identify his face and perceive the facial expression [3]. If the face is recognized, the position of the eyes is determined using 68 facial landmarks; based on these references, we define mathematically the centroid of each eye and the distance between them. In order to rotate the image, we’re obtaining an angle by drawing a straight line that crosses the two eyes and finally, the face is cropped, turned to grayscale consisting of 0 to 255 shades, and shrunk to 150×150 pixels. This way, the virtual “teacher” will be able to acknowledge whenever the student gets confused so that it’ll show a textbox asking, “Still need help?” and give further information if necessary. Also, with this feature, the learner’s eyes are tracked so that every time he looks away for more than 3-5 seconds, the app will show a warning to keep them focused. This is, in fact, one of the core values of our system, because by implementing a focus-assist procedure, we bring supplemental work in quality of learning experience, with visible long-term results.
To improve the accuracy of the algorithm, the students using this app can leave a review after receiving an answer from their virtual teacher.
Knowing today’s technology, a robot would manage to get to know a lot more about you than a human could possibly do, even though he hasn’t met you personally. Every click you make improves his algorithm and defines your personality so that the interaction becomes as “humanly” as possible. This way, each student using the app will have a unique experience due to their different personalities that the instructor needs to hone and work with in order to meet their learning needs.
The system also tracks how long it takes the students to respond to questions, keeps track of their grades and performance history, offers reports on their strengths, weaknesses, and motivation levels, and predicts their grades. The software adapts to each learner, identifying knowledge gaps and providing game-type quizzes to make studying more enjoyable.
At the end of their studies, undergraduates will still have to take a final exam in order to obtain a certificate of academic accomplishment, however without having crossed the world to attend university.
Vocal Assistance
Virtual teachers are designed to carry out their educating responsibilities; whatever knowledge you wish to pass on to the students in the class can be inputted into the digital mentor without restriction [2]. They are designed to know any language and can do more than a human is physically capable of; they are able to vocally deal with students’ requests in the second language, as well as play interactive games and even carry on a conversation. At first glance, this may not seem such an important factor. However, when you come to think of it, in a fully human interaction, both parties engage in the conversation equally; actually, in the case of student-teacher discussions, most of the talk is coming from teacher’s side. With a virtual teacher, the undergraduate might have a steadier conversation and be the initiator of actions, as in regular conversation. Furthermore, a non-human mentor won’t ever be sidetracked from the lecture he is conveying, surprised or take too long telling a story; it can’t lose its temper anytime while teaching or be biased against anyone. Henceforth, the class hours are effectively used, and the students will spend the study time to the fullest.
Now regarding the vocal assistance part, we’ll present the artificial intelligence behind ViTeach, used in order to offer the virtual teacher a more “human” impression. There is no doubt that audio is an amazing method to pass on data and subtext simultaneously. Truth be told, a speaker’s voice can give essential signals that help undergraduates with holding and recovering the data later. Audience members are more able to work with a voice – particularly in case it is clear, articulated and persuading – than they are to understand a text.
Huge amounts of data are required for technologies that support virtual teachers, which power artificial intelligence (AI) [9] platforms such as machine learning, natural language processing, and speech recognition. Since each of these processes involves somewhat a different kind of approach, we must study each aspect separately to create a better idea of the architecture behind which we would naturally call “artificial intelligence”. The term AI involves many fields of study that are focused on the development of human behavior in computing protocols. The purpose of AI is to create machines that act and think like humans, and the pursuit for such achievement is a matter of a rapidly growing global interest. However, the study goes even further, in a way that, artificial intelligence is only the bigger picture that involves all the subsets and processes previously mentioned. As we want to create more of a “humanlike” behavior, the algorithms’ complexities gradually increase, and simple decision-making commands are unable to solve the problem by themselves. The complexity of the algorithms can be highlighted by extending our study to other two important factors: machine learning and deep learning.
Machine learning is one of the bigger subsets and involves a rather straightforward approach that is quite useful when talking about the capabilities of our system. When a user interacts with a virtual teacher, the AI advanced algorithm learns from the inputted data and gets better at anticipating the user’s future demands. Machine learning algorithms create a model from sample data, referred to as “training data,” so as to make forecasts or choices based on experience, without being especially programmed to do so. As an example, if the student seems to be interested in Taylor Series by searching these specific words constantly, the software will remember this preference in the future and will also show him articles about Fourier Series or any related studies. The architecture behind such algorithms involves decision-making panels with singular inputs and multiple outputs. What is necessary to keep in mind is the machine learning method, which is highly influenced by the human factor. Generally, the experience is gained through trial-and-error interactions, therefore, by introducing human-assisted decisions, there are more chances that performance and accuracy are greatly increased. Through this learning method, which we would call supervised, the most important factor is the human element, which provides necessary feedback for the algorithm output. In our scenario, the training data are generated through historical data, like web search engines. The logic behind suggesting similar results is explained both at a local and global level through multiple mathematical aspects (such as Boolean algebra, conditional probabilities, distributions, frequency functions, likelihood tests), ranking systems, and structured query language. Once the training data are generated, the user can send positive or negative feedback regarding the usefulness of the suggestion, that would directly influence the decision-making protocol for the next set.
Another aspect we are considering in building such an application is the NLP (natural language processing) [10] subset which allows the virtual teacher to comprehend natural language in the same way that a human would do. NLP employs artificial intelligence to get real-world information, interpret it, and adjust it in a way that the virtual mentor can comprehend, whether the language is spoken or written. For the information to travel from the student to his digital “teacher”, data preprocessing and algorithm development are needed: data preprocessing sets up text information by highlighting features that an algorithm can work with; then the algorithm (e.g., Rules-based system, Machine learning-based system etc.) is developed to process it.
The virtual teacher is also using speech recognition [11], also known as speech-to-text, which means that it is able to recognize words spoken aloud and convert them into written language. Because rudimentary speech recognition technology has a restricted vocabulary, it can only recognize words and phrases once they are spoken clearly. However, the advanced software of ViTeach would handle natural speech, various accents, and different languages by following these four steps: inspect the audio; segment it; change it into a computer-readable format; and then use an algorithm to pair it with the most appropriate text representation. The virtual mentor is trained on different speaking manners, dialects, accents, languages and speech patterns. It also distinguishes human voice from the often-present background noise. When it comes to language learning, the teacher will use speech recognition software to recognize the student’s voice and provide pronunciation assistance. Furthermore, it may analyze specific voice features to detect the learner’s emotional state so that, when combined with the facial expression recognition we presented above, it can indicate how a person feels about the lesson.
Describing such applications allows us to step further into our final understanding of AI by studying deep learning models. Keeping in mind the ML functionalities, deep learning is not only a subset of AI but is also perceived as a machine learning part since the building principles follow similar approaches. However, perhaps the most important advantage that deep learning brings to computational thinking is the ability to solve complex problems, adapting to large volumes of data and many particular cases, which traditional ML algorithms are unable to process. Two such complex problems (NLP and speech recognition) were highlighted, and whilst explaining them in natural language is necessary, we must look into the actual functional parameters that serve as the engines of the processes. The main aspect regarding deep learning algorithms that we must consider is the mathematical background they use because as the problem gets harder and its answer more uncertain, conventional solutions’ usefulness decreases. One example from the real world is predicting an object’s trajectory, which is a rather simple problem, having known certain parameters (ex. position and speed). However, when extending at a quantum level (particle behavior), general laws do not apply anymore, so the answer becomes probabilistic. Thus, it is essential to treat complex computational problems by using deterministic approaches, and this is exactly what deep learning inquires.
The logic behind deep learning algorithms sits in the proximity of graph theories and special mathematics, where distributed representations such as neural networks, support vector machines and logistic regressions are indispensable tools. In our case, both NLP and speech-to-text are processes that can greatly benefit from neural networks’ applications, which are designed in such a way that they highlight the most probable outputs, being given a large set of inputs. In trivial terms, a neural network is a black box with an input which can be, in our case, the written message in natural language, or the audio spectrogram of dialogue, and an output that can be the analyzed word embedding. In mathematical terms, a neural network is a function with parameters that undergo a series of transformations, bypassing a large number of conditions through multiple layers. The output of our function should contain a dataset of desired parameters based on probability and likelihood tests. Recurrent neural networks (RNN) are relevant approaches since they process the information on the basis of some previously defined conditions (depending on the results obtained), and their variants such as gated-recurrent networks or long short-term memory networks were our choice as best-fitting for our operations. The discussion about the roots of the algorithms and the protocols within the layers of a neural network is as important as the hardware power needed for memory transfer, and each part can be a subject of discussion for further studies, nevertheless, our intention was to isolate the fundamental composition in order to explain the more general characteristics as key aspects in understanding the engineering standards behind our proposed work.
Conclusion
As the technology is rapidly advancing, the way users educate themselves is changing from the traditional blackboard to learning in an online environment [4]. In the last years, Internet apps have been more inclined towards enabling distance learning, as University students all across the globe utilize them for academic and research work. We also provided a survey which, in the end, proved the necessity of a reliable source of scientific information, since students feel a lot more comfortable talking to their virtual instructor than asking the school teacher. The study found that almost 90% of the students are already choosing Google over their teacher for school homework, since over 65% have felt ashamed to ask their mentor a question at least once.
This paper is taking a step forward and indeed bringing technology to the next level, introducing the concept where students can learn electronically through ViTeach – an e-learning platform for any age/level of study – hence breaking the barriers across the world. The app would include 5 general options to choose between when opening the menu: Home, Classes, Chat, Suggestion box, and Do not disturb. From there, the student can browse freely and find any piece of information he needs. He can also interact with other undergraduates, join study groups or discussions, share ideas, all behind an avatar. By communicating through avatars – computerized substitutes for themselves – the students’ shyness will be fundamentally decreased and it will encourage open thinking. Just as in real world, the app will be able to monitor undergraduates’ actions and act accordingly to improve the efficiency of its algorithm. Since the ViTeach website also covers practical areas besides the scientific ones, our future plan is to extend the survey and gain a wider range of opinions from universities such as arts, sports or even theatre and cinematography.
References
- ·Botelho, B. (2017), ‘Virtual assistant (AI assistant)’, TechTarget [online]: https://searchcustomerexperience.techtarget.com/definition/virtual-assistant-AI-assistant
- Dhawan, S. (2020), ‘Online Learning: A Panacea in the Time of COVID-19 Crisis’, Journal of Educational Technology Systems, 49(1), 5–22: https://journals.sagepub.com/doi/full/10.1177/0047239520934018
- Jaligama, V. and Liarokapis, F. (2011), ‘An Online Virtual Learning Environment for Higher Education’, Third International Conference on Games and Virtual Worlds for Serious Applications, 2011, pp. 207-214: https://www.researchgate.net/publication/224249396_An_Online_Virtual_Learning_Environment_for_Higher_Education
- Lutkevich, B. and Burns, E. (2021), ‘Natural language processing (NLP)’, TechTarget [online]: https://searchenterpriseai.techtarget.com/definition/natural-language-processing-NLP
- ·Lutkevich, B. and Kiwak, K. (2021), ‘Speech recognition’, TechTarget [online]: https://searchcustomerexperience.techtarget.com/definition/speech-recognition
- Newton, D.P. and Newton, L.D. (2019), ‘Humanoid Robots as Teachers and a Proposed Code of Practice’, Frontiers in Education [online], vol. 4: https://www.frontiersin.org/articles/10.3389/feduc.2019.00125/full
- Rahman, H. (2020), ‘Exploring the Students’ Attitudes toward Internet Usage for Academic Excellence’, The International Technology Management Review, 9(1), 58-63: https://www.researchgate.net/publication/343710006_Exploring_the_Students’_Attitudes_toward_Internet_Usage_for_Academic_Excellence
- Ramis, S., Buades, J.M. and Perales, F.J. (2020), ‘Using a Social Robot to Evaluate Facial Expressions in the Wild’, MDPI, Sensors 2020, 20, 6716: https://mdpi-res.com/d_attachment/sensors/sensors-20-06716/article_deploy/sensors-20-06716-v2.pdf
- Rose, M., ’Is Distance Learning Lacking In Communication?’, Street Directory [online]: https://www.streetdirectory.com/travel_guide/14560/education/is_distance_learning_lacking_in_communication.html
- Sabah, N.M. (2013), ‘Students’ Attitude and Motivation Towards E-learning’, First International Conference on Applied Sciences, 2013, vol. Gaza-Palestine: https://www.researchgate.net/publication/257984303_Students’_Attitude_and_Motivation_Towards_E-learning
- Stanislav, H.I. (2016), ‘Will robots substitute teachers?’, 12th International Conference ‘Modern science, business and education’, 2016: https://www.researchgate.net/profile/Stanislav-Ivanov7/publication/304490445_Will_robots_substitute_teachers/links/57711de708ae6219474a35f8/Will-robots-substitute-teachers.pdf