Introduction
The question of whether larger (and/or older) firms are more profitable than their smaller counterparts has been in interest of numerous researchers for decades. Large amounts of theoretical as well as empirical research in different disciplines (economics, strategic management, finance) have been conducted in order to demystify this relationship. However, while some authors found a positive influence of firm size on its performance, the others found this relationship to be negative or non-existent. With regards to the firm age, the interest in this variable began to grow in parallel with the studies that included firm age as an explanatory factor in investigations of determinants of firm performance i.e. business success. Although some theoretical explanations regarding the higher/lower level of profitability achieved by older firms are offered, due to the scarcity of empirical researches, no clear cut exists. One of the possible reasons for paucity of studies on this topic could be found in the absence of relevant data about the year of firm incorporation. Therefore, in order to exploit the benefits of Amadeus database and to give our contribution to the existing literature on this subject, in this research a role of firm age on business success is investigated on a panel of firms operating in the Croatian food manufacturing industry in the 2005-2014 period.
According to The Institute of Economics, food manufacturing industry has achieved the largest share in the gross domestic product and total employment, when compared to other manufacturing sectors in Croatia in 2014 (Ekonomski institut, 2015). More accurately, Croatian food industry has a long tradition and recognizable brands, and makes 28.2% of total production and 20% of total employment of manufacturing industry. The share of food industry in GDP in 2012 amounted to 3.2%. At the same time, the share of food production in GDP of the manufacturing industry accounted for 21.8%. Furthermore, the food industry in 2014 accounted for 8.8% of the total exports of manufacturing industry, and 7.7% in the total exports of the Republic of Croatia. When compared to the previous quarter, a production activity of this industry at EU-28 has increased by 0.43% in the last quarter of 2014 (Ekonomski institut, 2015). All these numbers clearly indicate the importance and significance as well as the high potential that the Croatian food industry has, which as such should be recognized as the strategic industrial sector of the Croatian economy.
The rest of the paper is organised as follows. An overview of the previous studies and their findings relating to the content being analysed is provided in section 2. Section 3 presents data, research methodology and variable description, while section 4 gives insight into the sample description and econometric model including the empirical results. Section 5 presents concluding remarks.
Literature Review
While some theoretical models considered the firm size as linearly related to firm age (Greiner, 1972), the others postulated specific predictions regarding the influence of firm age on its performance. Coad et al. (2013) summarized these predictions in three categories: selection effects, learning-by-doing effects, and inertia effects, depending on whether firm performance (expressed as a firm productivity) remains the same, increases or decreases over time. In empirical sense, firm age has been researched in different contexts. Starting with the influential work of Gibrat (1931) and finding that smaller, younger firms are more likely to grow faster than larger, older firms (in terms of the number of employees or amount of sales), a large number of researches have tried to explore the relationship between firm size and growth rate (see for example, Babirye et al., 2014; Bentzen et al., 2012; Palestrini, 2007; Evans,1987), as well as the relationship between firm age and growth rate (e.g. Barba Navaretti et al. 2014; Carr, 2010). While former relationships have usually turned out to be negative, no clear–cut has been made between firm age and survival (e.g. Bartelsman et al., 2005; Farinas and Moreno, 2000). Recently, some researchers were exploring a moderating effect of firm size or age on the relationship of analysed variables of interests like for example, relationship between institutional quality and export performance (LiPuma, 2013), relationship between organizational innovation, learning and performance (Hui et al., 2013) or relationship between R&D investment and firm performance (Fortune and Shelton, 2014), etc. Apart from the studies that were analysing a moderating effect of age in different industries/countries simultaneously, there were also studies that were concentrated on one specific industry (e.g. Ismail et al., 2014). A certain number of researchers have also examined differences in firm performance (profitability and/or productivity) at different stages of age.
Since the main focus of this research is oriented toward investigation of the nature of the relationship between firm age and its profitability, a brief presentation of recent empirical studies from this path of research follows. In order to observe whether older firms are more profitable because of their size or there is an age effect that can be revealed while controlling for firm size, Coad et al. (2013) explicitly included size and age variables in regression equation. With the application of different methodologies (OLS, LAD, VAR) on the panel of Spanish manufacturing firms operating between 1998 and 2006, the authors found the evidence supporting both, improvements of firms with age (i.e. it was shown that the older firms were experiencing rising levels of profits, productivity, larger size, etc.) and deteriorations of firms’ performance with age (the results also revealed lower profitability when other variables were controlled for; lower expected growth rates of profit, sales and productivity). On a sample of 1,020 Indian firms, Majumdar (1997) investigated whether the size and age of firms impacted firms’ profitability and productivity. According to this author the age-performance relationship is environment-specific and dependent on a number of institutional factors, and hence cannot be analysed outside the institutional framework within which firms operate. The results of the conducted analysis showed that the older firms were more productive and less profitable, while the larger firms were more profitable and less productive. With the application of the maximum likelihood ordered logit estimates with robust standard errors, on a sample of 58,211 manufacturing and services firms observed over the period 2004-2012, Bruni et al. (2014) found a significantly negative sign of age variable on EBITDA to sales ratio.
Some researchers, in line with our study, focused their attention on only one particular industry. Bhayani’s research covered all the listed cement firms operating in India during the period from 2001 to 2008 (Bhayani, 2010). With the utilization of the backward regression analysis, the results showed that the age of the firm (positive sign), together with some other internal and external variables (liquidity, operating profit ratio, interest rate and inflation rate), played a vital role in the determination of the firm profitability in Indian cement industry. Opposite to this study, on a sample of 57 business group companies of Indian nonmetallic mineral products industries, for the10-years period, Gaur (2011) examined the influence of different variables, among which the age variable as well, on operating profit and return on net worth, however, the age variable didn’t prove to be statistically significant. Summarizing the results of the presented studies, it becomes clear that the empirical researches regarding the relationship between the firm age and profitability remain equivocal. Although a progress has been made in the investigation of firm age on its performance, as noted by Coad et al. (2013), there are still many opportunities remaining for improving our understanding of how firm performance/behaviour changes as firms grow older.
Data, Research Methodology and Variables
The data for the research were collected from the AMADEUS database compiled by Bureau van Dijk. Since AMADEUS provides information at the 4-digit (NACE Rev. 2) level, the sample was created by including all firms recorded in any 4-digit NACE Rev. 2 food processing industry (categories between NACE-1011 and NACE-1099) for the period from 2005 to 2014. This industry was chosen due to its significant contribution to total manufacturing industry in terms of total production (28.2%), total employment (20%), and value of industrial production sold (17.6%), as well as due to the overall data availability.
AMADEUS has the advantage that it comprises firms of all possible sizes. This is an important issue since 95.3% of all firms operating in the EU food and drink industry are micro and small sized firms (FoodDrinkEurope, 2014). Therefore, unlike previous studies that were restricted to publicly quoted firms or that have used some minimum firm size criteria while constructing the research sample, the present study encompasses micro and small sized firms together with the medium-sized, large and very-large companies. Given that the total number of analysed firms was changing over the years (as a result of the entering of new firms in the market, mergers, acquisitions and liquidations), we were dealing with unbalanced panel (number of times-series observations was different across firms). After imposed restriction according to which only those companies that were active three consecutive years can constitute the sample, the total number of firms included in the analysis amounted to 956.
Descriptive statistics for all variables used in the analysis is shown in Table 1. The industry average is provided by mean along with the variables’ minimum and maximum for period covered by analysis, while standard deviation indicates the interindustry variation of the variables value within the 2005-2014 period.
Table 1: Descriptive statistics
Source: Authors` calculations
Many of the economic relationships are dynamic in their nature and therefore, in order to evaluate the influence of firm age on its business success, we introduce a dynamic component into the model (1). Since lagged dependent variable also appears as an explanatory factor in the model, it correlates with the error term and strict exogeneity of the regressors no longer holds. Estimating model (1) via OLS would yield biased and inconsistent results. In order to overcome this problem and to obtain consistent estimator of , the authors used generalized methods of moments (GMM) panel estimator developed for dynamic panel models by Arellano and Bond (1991) and Arellano and Bover (1995). Discussion and various estimation methods for dynamic panel data are given by Baltagi (2005). Two-step estimator is employed on the following model:

where πit is the profitability of firm i at time t, with i=1, . . ., N, t=1, . .., T; α is a constant term, πi,t-1 is the one-period lagged profitability, δ is the speed of adjustment to equilibrium, Age stands for firm age, is the parameter of the variable of our interest – firm age, is the vector of coefficients to be estimated, is a set of the control variables, εit is the disturbance, with νi the unobserved firm-specific effect and uit the idiosyncratic error.
All variables used in the analysis are chosen on the basis of relevant theory and empirical literature. The description of the chosen variables (i.e. EBITDA Margin, Age, Size, Liquidity, Solvency and Gearing) together with their expected influence on the firms’ profitability is given in the following sections.
As a dependent variable (measure of profitability), we used EBITDA Margin which is calculated as a ratio of the company’s Earnings Before Interest, Taxes, Depreciation and Amortization, on one side, and its operating revenue, on the other side.
Age is used as a main explanatory variable in model (1). Since the focus of the present paper is put toward this variable, it is of interest to analyse and confront different theoretical aspects of the relationship between firm age and its performance. In theoretical discussion on age-performance relationship, the arguments can be found for both, positive and negative relationship. Argument for positive influence of age on performance lies in firm experience, since older firms can benefit from accumulated knowledge in all crucial aspects of the business (better technology, well-developed supply channels, well established customers’ relations, easier access to resources, better human capital and lower financing costs). As older firms have more experience, abilities and skills, and since they have enjoyed the benefits of learning, they consequently can enjoy superior performance. Although previously described arguments are quite convincing, so are those that stream to explain a negative influence of firm age on performance. As firms become older, they often try to codify decision making procedures, what makes them very bureaucratic, reduces organizational flexibility and ability for prompt changes. Rigid rules and procedures can be very large obstacles for organizational changes and innovation which are crucial in the modern globalized and very competitive business environment. Also, with the age firms might pursuit the strategy of “quiet life” and consequently avoiding risks (large R&D investments), large restructuring, conflicts with employees, etc. Avoidance of organizational changes and R&D investments in long term results in losing the competitive advantages and decreased performance. In line with this reasoning, a negative influence of this variable on profitability is anticipated. Age of the firm is expressed by the number of years that the firm operates in the market.
Control variables. In order to control for different firms’ characteristics, a set of control variables is introduced in the analysis. Each of the control variables captures a specific aspect of firms’ activity. Thus for example, Size variable had task to control for firm size, since the size of a firm can serve as a source of economies and diseconomies of scale (Besanko and Braeutigam, 2011), therefore both, positive or negative sign of this variable may appear. Liquidity represents firm’s ability to settle short-term liabilities resulting mainly from operating costs. Current liquidity is often calculated as the ratio of current assets and current liabilities. The firm should maintain required level of current assets in order to cover current liabilities and according to theoretical consideration the optimal ratio of this variable should equal 2. Alternative measure of liquidity, the quick liquidity (Current Assets-Stocks/Current Liabilities), which is used in this research, should be at least 1. Liquidity below ideal values indicates that firm is not liquid and consequently cannot settle its current liabilities. Illiquidity is often the consequence of poor operations and can be related with lower firm performance. On the other hand if the firm overinvests current assets, it can also negatively affect business success due to higher financing costs in overinvested current assets. Having in mind previously described characteristics of liquidity, this variable can have both, positive and negative influence on firm performance. Solvency describes the firm’s financial health and capacity to repay long-term liabilities. In line with theoretical aspects, shareholders’ funds should cover at least 50% of total assets. If shareholders’ funds fall significantly below 50%, firms’ risk of default/bankruptcy rises, what is often reflected in higher financing costs and lower performance. Therefore, it is to expect that the higher solvency should result in higher performance, i.e. a positive sign of solvency variable is to be anticipated. Finally, the Gearing ratio measures the structure of financing sources, i.e. ratio of long term borrowings and shareholders’ funds. Firm growth often requires financing by long term debt which increases gearing and firm risk. Namely, highly leveraged firms are perceived as being more risky, since in period of economic downturn, the firm must repay its debts regardless of negative trends in sales and cash flows. Without long term debt, firms could not achieve the required level of investments and therefore certain level of gearing is necessary. Therefore, financial managers in firms should achieve optimal level of gearing, while having in mind that too high level of gearing might significantly increase insolvency/bankruptcy risk and financing costs. On the basis of the previous discussion, we expect that gearing negatively affects firm performance.
Summary of the variables and corresponding measurements is presented in Table 2.
Table 2: Measurement of Variables
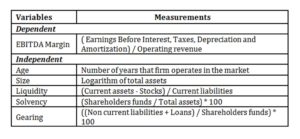
Results and Discussion
Pairwise correlation matrix is shown in Table 3, while Table 4 presents the results of the dynamic panel analysis estimated using two-step General Method of Moments (GMM) as proposed by Arellano-Bond. Most of the correlation coefficients demonstrate weak correlation between variables. The highest value of the coefficient is obtained between Solvency and Gearing variable and amounts to -0.5087, which is still far below the acceptable level and the possibility that problem of multicollinearity occurs.
Table 3: Pairwise correlation
Source: Authors` calculations
For consistent estimation, GMM estimator requires that the error is serially uncorrelated. First-order and second-order serial correlation in the first-differenced residuals is tested using m1 and m2 Arellano and Bond test statistics. The GMM system estimator is consistent if there is no second-order serial correlation in residuals (m2 statistic). This means that the presence of a first-order autocorrelation in the differenced residuals does not imply that the estimates are inconsistent (Anderson and Hsiao, 1981). Another important test in dynamic panel analysis is Sargan test. This test checks for the overall validity of instruments. If a null hypothesis is accepted, meaning that overidentifying restrictions (all chosen instruments) are valid, the dynamic panel model is adequately specified.
Table 4: Parameter estimates of dynamic panel model
Source: Authors` calculations
With regard to the statistical tests, empirical results obtained for the model (1) show no presence of second-order autocorrelation. Likewise, Sargan test shows no evidence of over-identifying restrictions. This suggests that the dynamic panel model is adequately specified. Furthermore, table 4 reveals that lagged profitability variable (EBITDA Margint-1) has statistically significant influence on the current firms’ profitability which confirms the dynamic character of the model specification. Regarding the main variable of our interest (Age), it can be perceived that, after controlling for other influences, firm age has statistically significant negative influence of firm’s performance. This finding indicates that for the firms operating in the Croatian food manufacturing industry, one can expect deterioration of firm performance as time goes by. This finding is opposite to that of Bhayani (2010), but in line with those of Majumdar (1997), Coad et al. (2013) and Bruni et al. (2014), and can be interpreted in a way that due to bureaucratic ossification older firms become inert, without required flexibility to adapt to new business circumstances and therefore they are likely to be outperformed by younger, more flexible firms.
It should be noted that selected control variables (except Gearing) have statistically significant influence on firm performance i.e. business success. The sign of the coefficients of all control variables are in line with our expectations. More precisely, positive sign of Size variable indicates that firms may exploit economies of scale and scope, and therefore benefit as becoming larger. Also, firms with higher Solvency perform better, pointing that they are perceived as being less risky and therefore can operate with lower financing costs. The results also indicate that firms with lower Liquidity achieve better performance, i.e. strategy of minimizing working capital can increase the firm performance. Finally, although the negative sign of the coefficient of Gearing variable indicates that increased leverage reduces performance, this variable is not statistically significant. In general, apart from the fact that the coefficient signs of the explanatory variables are in line with the expectations, they are also comparable with findings from previous studies (Pervan, 2013; Vijayakumar, 2011; Obert and Fatoki, 2010; Feeny et al. 2005).
Conclusion
The aim of this research was to determine the extent and nature of the relationships (if any) between firm age and business success. The analysis is conducted on firms operating in the Croatian food processing industry for the period from 2005 to 2014. The total number of firms included in the analysis amounted to 956. Given that the total number of analysed firms was changing over the years (as a result of entering of new firms, the mergers, acquisitions and liquidations), an unbalanced panel is formed. Food processing industry was chosen due to its significant contribution to the total manufacturing industry in terms of total production (28.2%), total employment (20%), and value of industrial production sold (17,6%) as well as due to the overall data availability.
The results of the conducted dynamic panel analysis reveal that age of firms is an important explanatory factor in the determination of business success. Precisely, on a sample of the Croatian food processing industry, firm age turns out to be statistically significant with negative coefficient sign, thus suggesting deterioration of firm performance with age. As firms grow older, they become very bureaucratic, with reduced organizational flexibility and ability for prompt changes. Also, firms might pursuit the strategy of “quiet life” and consequently avoid risks, large restructuring, conflicts with employees, etc. As regards the control variables that have the task to capture different firms’ characteristics, the results of the conducted analysis indicate a positive and significant influence of firm size and solvency on business success, as well as significant and negative influence of liquidity. Gearing variable didn’t prove to be statistically significant.
Overall, the present study enriches our understanding of firm age and performance in the Croatian food manufacturing industry and highlights the importance that some other variables, aside from age, may have on business success. From a manager policy perspective, the obtained results provide guidelines in the formulation of adequate business strategies and point to factors that managers must take into account in order to achieve better firm performance. Further researches may be oriented toward the investigation of the presence/existence of non-linear age-performance relationship, with its application on a broader research sample together with the inclusion of additional explanatory/control variables.
Acknowledgment
This work has been fully supported by the Croatian Science Foundation under the project UIP-2014-09-1745. Any opinions, findings, and conclusions or recommendations expressed in this material are those of the authors and do not necessarily reflect the views of the Croatian Science Foundation.

(adsbygoogle = window.adsbygoogle || []).push({});
References
- Anderson, T. W. and Hsiao, C. (1981), ‘Estimation of Dynamic Models with Error Components’, Journal of the American Statistical Association, 76(375), 598-606.
- Arellano, M. and Bond, S. (1991), ‘Some Test of Specification for Panel Data. Monte Carlo Evidence and Application to Employment Equations’, Review of Economic Studies, 58(2), 277-297.
- Arellano, M. and Bover, O. (1995), ‘Another look at the instrumental variable estimation of error-components models’, Journal of Econometrics, 68(1), 29-51.
- Babirye, S., Niringiye, A. and Katerega, E. (2014), ‘Firm Size and Rate of Growth of Ugandan Manufacturing Firms’, Journal of Applied Economics and Business Research, 4(3), 178-188.
- Baltagi, B. H. (2005), Econometric Analysis of Panel Data, Third edition, John Wiley & Sons Inc.
- Barba Navaretti, G., Castellani, D. and Pieri, F. (2014), ‘Age and Firm Growth: Evidence from Three European Countries’, Small Business Economics, 43(4), 823-837.
- Bartelsman, E., Scarpetta, S. and Schivardi, F. (2005), ‘Comparative analysis of firm demographics and survival: evidence from micro-level sources in OECD countries’, Industrial and Corporate Change, 3, 365-391.
- Bentzen, J., Madsen, E. S. and Smith, V. (2012), ‘Do Firms’ Growth Rates Depend on Firm Size?’ Small Business Economics, 39(4), 937-947.
- Besanko, D., Braeutigam, R. R., (2011), Microeconomics, Fourth Edition, John Willey & Sons, Hoboken.
- Bhayani, S. J., (2010), ‘Determinant of Profitability in Indian Cement Industry: An Economic Analysis’, South Asian Journal of Management, 17(4), 6-20.
- Bruni, S., Pittiglio, R. and Reganati, F. (2014), ‘Heterogeneity in Firm Performance During Economic Crisis’, Business, Management and Education, 12(1), 1–14.
- Carr, J. C., Haggard, K. S., Hmieleski, K. M. and Zahra, S. A. (2010), ‘A study of the moderating effects of firm age at internationalization on firm survival and short-term growth’, Strategic Entrepreneurship Journal, 4(2), 183-192.
- Coad, A., Segarra, A. and Teruel, M. (2013), ‘Like milk or wine: Does firm performance improve with age?’, Structural Change and Economic Dynamics, 24, 173-189.
- Ekonomski institut, (2015), Sektorska analiza, Hrana i piće, Zagreb, 37(4).
- Evans, D. S., (1987), ‘The relationship between firm growth, size and age: estimates for 100 manufacturing industries’, Journal of Industrial Economics, 35(4), 567-581.
- Farinas, J., Moreno, L., (2000), ‘Firms’ growth, size and age: a nonparametric approach’, Review of Industrial Organization, 17(3), 249-265.
- Feeny, S., Harris, N. M. and Rogers, M. (2005), ‘A dynamic panel analysis of the profitability of Australian tax entities’, Empirical Economics, 30(1), 209-233.
- Fortune, A., and Shelton, M. (2014), ‘Age Matters: Disentangling the Effect of R&D Investment in the Global Chemical Products Industry’, Business Management Dynamics, 3(11), 35-54.
- FoodDrinkEurope, (2014), ‘Data & Trends of the European food and drink industry: 2013-2014’ FoodDrinkEurope. [Online] [Retrieved March 10, 2016]
- http://www.fooddrinkeurope.eu/uploads/publications_documents/Data_and_Trends_2014-20151.pdf.
- Gaur, J. (2011), ‘Financial Performance Measures of Business Group Companies: A Study of Indian Non-Metallic Mineral Products Industries’, The IUP Journal of Business Strategy, 7(4), 45-53.
- Greiner, L. (1972), ‘Evolution and revolution as organizations grow’, Harvard Business Review, 76, 37-46.
- Hui, H., Jasimahbt, C. W., Radzi, W. M., Jenatabadi, H. S., Kasim F. A. and Radu, S. (2013), ‘The Impact of Firm Age and Size on the Relationship among Organizational Innovation, Learning, and Performance: A Moderation Analysis in Asian Food Manufacturing Companies’, Interdisciplinary Journal of Contemporary Research In Business, 5(4), 166-174.
- Ismail, N.A. and Jenatabadi, H.S. (2014), ‘The influence of firm age on the relationships of airline performance, economic situation and internal operation’, Transportation Research Part A, 67, 212-224.
- LiPuma, J. A., Newbert, S. L. and Doh, J. P. (2013), ‘The effect of institutional quality on firm export performance in emerging economies: a contingency model of firm age and size’, Small Business Economics, 40(4), 817-841.
- Obert, M. and Fatoki, O. (2010), ‘Does debt really matter on the profitability of small firms? A perspective on small manufacturing firms in Bulawayo, Zimbabwe’, African Journal of Business Management, 4(9), 1709-1716.
- Palestrini, A. (2007), ‘Analysis of Industrial Dynamics: A Note on the Relationship between Firms’ Size and Growth Rate’, Economics Letters, 94(3), 367-371.
- Pervan, M. and Mlikota, M. (2013), ‘What Determines the Profitability of Companies: Case of Croatian Food and Beverage Industry’, Ekonomska istraživanja, 26(1), 277-286.
- Vijayakumar, A. (2011), ‘The Determinants of Profitability: An Empirical Investigation Using Indian Automobile Industry’, International Journal of Research in Commerce and Management, 2(9), 58-64.