Introduction
One of the main objectives of all the governments consists in taking measures to reduce unemployment and also in making sure that most of the available workforce in the country is engaged in taxable activities. In order to achieve those objectives there is a constant need for relevant and accurate data that will enable the government institutions to both take informed decisions and to measure the impact of the past macro-economic policies.
However, when it comes to informed decision making, especially inside state owned agencies, there is often the issue of having to deal with databases and systems that are either incompatible, unsynchronized, un-linked or all of the above taken together. Moreover, there is a known reticence between agencies to collaborate with each other for various reasons. The fact that most of the state institutions have their own budgets and autonomy to implement the IT systems they see fit, without having to synchronize with other possible involved parties (like other ministries or congruent agencies that are having activities in intersecting or same fields) only complicates the matter at a macroeconomic policy level (see, among others, Popescu and Roman, 2018; Pirciog et al., 2015; Militaru and Cristescu, 2017; Roman and Popescu, 2015; Avram et al., 2013).
This is why, the main purpose of this paper consists in presenting a solution to deal with these issues by adopting a comprehensive set of identity management maintenance steps, including data governance, data migration and unified reporting mechanisms so that the decision makers can, in fact, have some sort of reliable datasets about the population and the impact of macroeconomic policies.
The proposed comprehensive set of identity management maintenance steps will be presented further below, by considering the logical data flow from recurrent and continuous data governance activities to data management practices and reporting mechanisms. Thus, the structure of the paper is the following: section 2 is dedicated to the cross-project (nation-wide program) data governance flow, while the data migration / transformation flow will be presented in section 3. Section 4 describes the reporting and data analysis process, while section 5 deals with Cloud access and identity management for government agencies. Finally the last section concludes.
The Data Governance Flow
The reason that always comes to data program wide governance practices is the fact that state IT systems are usually very vast and under constant state of change. This means that if one needs to have a good overview over individual identities (companies, persons, employees, etc.) with data spread across multiple IT systems, one needs to make sure that this data is always aligned as per agreed principles.
So as to meet governmental strategic and operational goals in the field of maintenance of identity (ID) management (Popescu et al., 2016), the government agencies should implement nation-wide data governance programs. This higher function should be established so as to provide control over high level data management tasks. At program level, the governance functions and processes are very distinct from the usual functional management activities (e.g. projects taken at the individual level, non-project work related activities and eventual subprograms) (see fig. 1).

Fig. 1: Data Governance Program Organization
Source: authors’ own adaptation from PMI (2016)
Data governance at program level should focus on decision making guidelines for general ID management tasks so that it can deliver the value needed to meet national strategic and operational goals – like lowering the unemployment rate by a targeted margin. This is very important at the macro-economic level, as the results can be in turn used not only to estimate and measure, but also for publicity and image purposes in future election campaign activities.
As defined by the PMI (2013) a program is “a group of related projects, subprograms and program activities that are managed in a coordinated way to obtain benefits not available from managing them individually. Data governance programs comprise various components, primarily subprograms and individual projects within the program. Non-project components or program activities are needed to manage the program itself such as training, operations, and maintenance.”
The “program governance” refers to the sum of the elements that comprise the governance activity, each having functions, processes and sub-programs (including implementation projects). The governance function means, basically, grouping processes related to each other and across governance domains in order to support the portfolios, the programs and the projects that the government wants to implement.
All of these functions are structured as control, integration, oversight and decision making activities (Popescu et al., 2017). The reason why the data governance activity is to be taken into account is that one needs some sort of overseeing body to manage cross-organizational identity data (in our specific case a program will always be meant to obtain a unified view over data related to entire classes active populations). In this way there is always a degree of control over what is happening at cross platform level and integration between databases and systems remains feasible over long periods of time.
The Data Migration / Transformation Flow
In the process of maintenance identity management over a multitude of very large, national, state-wide systems, the normalization is a very complex process with exponential business complications. This is due to the fact that different state agencies implement systems that best serve their particular interests (like social security, medical insurance, active teaching systems and requalification programs, military drafting, census specific databases, electronic voting counters, etc.) One can imagine that usually the data dimensions will never be easily comparable between those systems.
In this section we will present a general case study using as an example data migration and transformation practices with SAP legacy specific systems from multiple sources into one new unified destination. A 6 Step approach for data normalization and migration will be further on described.
The data migration in complex identity management projects will usually follow several steps that are most of the time repeating themselves. All data is usually stored into a common dedicated destination source (called form here on data warehouse – DWH). Depending on the complexity of the task and the government objective, several other, intermediary steps can be added in-between. The list below describes the steps taken during the program in order to finalize the data migration process and to reach a unified data modelling and reporting process. Each step will be further on described in some detail level:
- Data Readiness Evaluation
- Data Normalization
- Data Extraction
- Validating Techniques
- Data Transformation and Loading Process
Data Readiness Evaluation
During the data preparation phase, there are a number of tasks that need to be done before real data migration takes place, activities like installation and configuration of the specific data normalization tools (in SAP case the Data Services tools), creation of the data migration design templates, SDS standards, and also the determination of the data objects in scope (for example all data related to income measurement). This has been captured in the data migration object list. Furthermore, in this phase, the high level data migration and cleansing approach have to be clearly stated and delivered.
For the installation and configuration of the SDS tools will be responsible the data warehouse (DWH). The DWH team will be responsible for data templates and finalizing of the data object list and the data migration along with the cleansing approach.
Table 1: Correspondence between activities, deliverables and responsible parties
Source: authors own contribution
Data Normalization
Data normalization is a repetitive task that will be done in close cooperation with all related governmental agencies that have source data. The actual normalization should fall under the program implementer’s activities. The DWH team will supervise the data cleaning process and even be involved in the program’s governance.
Before the start of the related data accuracy tests, the presumption is that the newly data placed in the DWH is 100% clean. Therefore verifications and normalization of data process is iterative until the data is accepted as being fully cleaned so it can be used for Identity Management analysis. In some countries, where data access can be done only by the government agencies themselves Identity Management and Maintenance is not even relevant since the population will never access these source systems and do interrogations or ask for online services according to their level (like printing the tax return forms, or asking for online reports on their personal financial statements and at-level taxation levels).
Data Extraction (from source systems) and Validating Techniques
Usually there are 2 sub-steps to be considered at this level:
Step 1 (fig. 2) is the extraction of relevant (raw) data from the source systems, after which they are stored in the staging tables in DWH. In this first step there is no specific logic, however, the data will be taken over from the source system in a 1:1 ratio. When the data gets extracted from the source system there is a Step 2 in the extraction process where all the validation will take place and the data will be stored in two kinds of tables:
- _ERR tables containing data from the staging tables that does not comply with the validity rules
- _MAP tables containing data from the staging tables that passed the validity rules and can now be used for reporting and analysis.
Data Transformation and Loading Process
The data transformation represents data enrichment, normalization and aggregation. It is a crucial step that will basically bring the data from several sources to a level that can be used in analysis, reporting and decision making. It is described in fig. 2 being associated with steps 4 and 5.
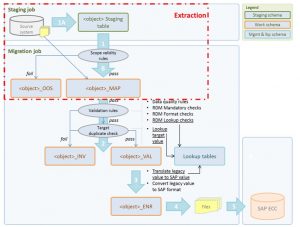
Fig. 2: Extraction process from multiple source systems
Source: authors own contribution
Reporting and Data Analysis
Having now all the Identity data with all the needed attributes linked to it, deep analysis activities can be performed by state institutes regarding aspects and patterns observed on the active population. Nationwide measures can be now taken using extensive unified reporting mechanisms that will try to help in the decision making process, implementation of combating poverty (see Avram and Militaru, 2016) and income inequalities (see Andrei et al., 2017; Popescu and Militaru, 2017; Zamfir and Mocanu, 2016; Cristescu, 2015; Matei et al., 2014), coaching and educational initiatives across entire classes of individuals (Roman and Popescu, 2015; Velciu, 2014; Pirciog et al., 2010) and so on.
There is, however, an aspect that is often neglected which regards the reporting process of the maintenance of ID management itself (and not the reporting on the impact and results of the governmental measures). With having controls implemented over the data, we propose a fully cyclic view that will close the circle and restart the entire process over and over. We are aware that over the years the data requirements will change, while more and more information will be needed to be linked to an identity. This means that with every cycle, during the governance phase, additional analysis and decisions need to be considered so as to meet the newly formulated requirements and challenges. This is achieved by implanting general metrics that will give an indication on how and what needs to be done and will show the current situation in regards to the entire set of data.
The scope of the article is not to go into detail over how data status is to be reported on, but as a program management recommendation that can be given. Thus, the following fields need special attention:
- Time needed to both get and analyze the data – For each system an allocated time needs to be put forward. This should include data analysis, transformation and loading.
- Data cost – This includes monetary values of both man-hours and other resources needed to get the needed data sets, but also hardware and software costs, legal fees and so on.
- Data quality (DQ) – On this we propose that a comprehensive set of metrics need to be taken into consideration that will reflect correctly the DQ status during all phases of the program
- Actions Needed – This includes not only the decisions taken, but also the time by which they need to be implemented, the responsible parties, the controlling entities and a detailed step-by-step description that will lead to the materialization of the objectives.
We propose that these points can be followed by using a combination of good practices and specialized tools like SAS and SAP reporting tools (see, for instance, Eslinger, 2016).
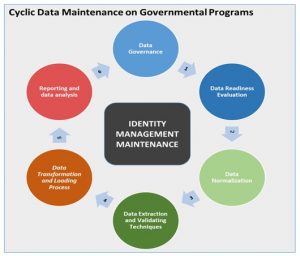
Fig. 3: The Data Maintenance Cycle
Source: authors own contribution
Cloud Access and Identity Management for the Government Agencies
In the digital era, connectivity can go a long way: tax payers nowadays expect at least to be able to be connected to the government agencies across all branches of the state apparatus and the state apparatus is increasingly dependent on connected devices and great amounts of cloud-based computational power to enable more efficient and competitive business models.
Hackers are also aware of the value that resides in Cloud and the Internet of Things and are increasingly targeting these state owned domains with attacks that can often have devastating consequences on state institutions – (like the attacks that happened two years ago at the US Homeland agency). Thus, in this context, Identity management becomes an enabler for leveraging all the technological advances that the Cloud and the Internet of Things can create with the least amount of risk.
All states recognize the Cloud and Internet of Things Security challenges as both opportunities and obstacles and addresses them by continuously drafting and creating a new security standards and regulations tailored for the taxpayer’s needs.
“The demand for agility in government IT programs makes cloud a natural fit, and for many agencies, the question isn’t whether to move to cloud, but what services can be provided more efficiently with less internal cost and resources. The continuing development of cloud computing requires government to create a road map and evaluate the steps that it needs to take now and in the coming years” (O’Brien, 2015)
Cloud adoption for the government agencies is sometimes nuanced since options are often considered by the public sector going to the essentially private owned cloud. Weighting cloud benefits and risks will, in the end, determine cloud adoption. Technology strategic planners have to understand all adoption nuances and will try to find the opportunities by capture cloud growth in government.
Government agencies are, however, not migrating to the cloud as quickly as foreseen—in fact, the U.S. Government Accountability Office, for example says agencies have increased their cloud investments by only 1 percent in the last half presidency term (about two years). Still, most state representatives from all around the world agree that moving to the cloud is critical to meeting growing demands and delivering public service for the future. So, why does it take so long?
Beyond all the budget and security constraints, agency cloud concerns have to include the manageability and lack of control aspects. How are they mitigated and will they bring a significant new management challenges? Bringing the development and operation facets together— the new DevOps (software development (Dev) and software operation (Ops)) trend— could well become part of the future solution.
Even as cloud solutions become ubiquitous across the Communications, Media and Technology landscape, the government organizations still struggle to optimize the technology’s adoption, largely because each ministry requires unique implementation and migration strategies (Sabău-Popa et al., 2015).
At times, governments have mistakenly pursued “migration-first” approaches when adopting cloud technology, viewing it simply like another hosting environment onto which they can transfer problematic all their internal legacy IT solutions.
By doing so, they can figure that they can cut on infrastructure costs and improve performance, but the initiatives are often short-circuiting in their cloud adoption process by allowing of “legacy first” routes and permitting influential and political factors to resist the change – instead of getting all the advantages of these new capabilities that this modern and highly elastic platform offers. Consequently, they miss on about 85 percent of the cloud’s functions.
By not focusing on the legacy constraints, the new cloud capabilities will offer the highest rate of flexibility and generate a gravitational pull within the state agency.
Several scenarios for cloud realization and IT optimization can be considered for the government organizations. Among the main major scenarios of how the government organizations could use cloud to drive change in their technology environment, we consider the following:
- Scenario 1. Exploring the new
- Scenario 2. Melding new and old platforms
- Scenario 3. Achieving purpose-driven data migration
- Scenario 4. Streamlining and optimizing legacy platforms
So, to satisfy the government general requirements for ID Management, we need to answer the question regarding what the main points are, considering all the above observations and particularities.
The ideal cloud-centric platform needs to be comprehensive from a security point of view and will need to have a fully integrated identity platform. It also has to provide hybrid identity features by supporting single identity pinpointing functions for a user across cloud based application and on-premises.
Moreover, it also needs to be based on multi-tenant and micro service architecture. Each functionality is, in fact, a micro-service which is independent of each other like Management, Provisioning, Analytics, and so on. And last but not least, it needs to be open to ever-changing government standards.
Finally, we propose a suggestion of short list of the mandatory requirements for such a platform:
- (SSO) – Single Sign On
- Identity and Access Management capabilities
- On-Premise AD/OAM Integration (Using IDCS as SP)
- User Authentication Service
- Identity Federation Service
- Token Service
- Reporting Service
Conclusions
Because analysis cross-government database system is such a resource intensive task, continuous data maintenance identity management is a must. This is achieved via program governance specific activities that should not change when there are changes at political level. The data maintenance process should be continuous and should have the objective of keeping the links between systems (unified keys, data transformation rules, relevant reports) always relevant.
Moreover, if this is achieved, then personalized government services to the population can be offered, since reaching and maintaining data unification cross systems will enable the population to log into the state systems from exposed interfaces and use their private IDs to perform various tasks like paying taxes online, filing complaints over the internet, checking tax situation from their mobile telephones, etc.
Since the analysis of cross-government IT systems is a very demanding task, continuous data maintenance on identity management becomes mandatory. Thus, we suggest achieving this via governance specific activities that should not be impacted by changes at the political level.
The use of cloud options can also be taken into consideration, as more and more governments are nowadays migrating to cloud as a last resort in keeping pace with technology changes and reducing IT maintenance. The data maintenance process should, therefore, never be stopped in order to insure the links between all the relevant systems.
Moreover, all these activities can be linked to specific persons, along with their other relevant data and based on these electronic identities, the government can take active measures, implement various programs, verify the results, launch internet campaigns and, in the end, help their citizens by taking informed decisions.
(adsbygoogle = window.adsbygoogle || []).push({});
References
- Andrei, A.M., Galupa, A. and Georgescu, I. (2017), ‘Measuring the influence of economic and social inequality on growth. Empirical evidences for Central and Eastern Europe’, Proceedings of the IE 2017 International Conference, ISSN 2284-7472, May 04 –07, 2017, Bucharest, Romania, 463-469.
- Avram, S., Figari, F., Leventi, C., Levy, H., Navicke, J., Matsaganis, M., Militaru, E., Paulus, A., Rastrigina, O. and Sutherland, H. (2013), ‘The distributional effects of fiscal consolidation in nine EU countries’, EUROMOD Working Paper EM2/13, University of Essex, Colchester.
- Avram, S. and Militaru, E. (2016), ‘Interactions Between Policy Effects, Population Characteristics and the Tax-Benefit System: An Illustration Using Child Poverty and Child Related Policies in Romania and the Czech Republic’, Social Indicators Research, 128(3), 1365-1385.
- Cristescu, A. (2015), The impact of the economic crisis on the regional disparities of earnings in Romania, The USV Annals of Economics and Public Administration, Vol. 15, 2(22), 34-41.
- Eslinger, J. (2016), ‘The SAS Programmer’s PROC REPORT Handbook: Basic to Advanced Reporting Techniques’, SAS Institute Inc., Cary, NC, USA.
- Matei, M.M., Zamfir, A.M. and Lungu, E. O. (2014), ‘A Nonparametric Approach for Wage Differentials of Higher Education Graduates’, Procedia – Social and Behavioral Sciences, 109, 188-192.
- Militaru, E. and Cristescu, A. (2017), Child policy changes and estimation of income distribution effects, Theoretical and Applied Economics, Vol. XXIV, 2(611), 187-196.
- O’Brien, A. (2015), ‘Business Strategy: Government Cloud Adoption — A Transformative Force Impacting IT? ‘, IDC Journal, (ID# GI258530)
- Pirciog, S., Ciuca, V. and Popescu, M.E. (2015), ‘The net impact of training measures from active labour market programs in Romania – subjective and objective evaluation’, Procedia Economics and Finance, 26, 339–344.
- Pirciog, S., Lungu, E.O. and Mocanu, C. (2010), Education-Job Match among Romanian University Graduates A Gender approach, Proceedings of the 11th WSEAS international conference on mathematics and computers in business and economics, ISSN: 1790-2769, June 13 – 15, 2010, Iasi, Romania, 205-210.
- PMI (2016), ‘Governance of Portfolios, Programs and Projects: A Practice Guide’, Project Management Institute, Newtown Square, PA.
- PMI (2013). ‘The Standard for Program Management’– Third Edition, Project Management Institute, Newtown Square, PA.
- Popescu, I.P., Barbu, C.A. and Popescu, M.E., (2016), ‘Identity and access management- a risk-based approach’, Proceedings of the 9th International Management Conference “Approaches in Organisational Management”, ISSN 2286-1440, 5-6 November 2015, Bucharest, Romania, 572-580.
- Popescu, M.E. and Militaru, E. (2017), ‘Wage inequalities in Romania under successive adjustments in minimum wage levels’, Theoretical and Applied Economics, 2 (611), 213-220.
- Popescu, M.E., Popescu, I.P. and Barbu, C.A. (2017), ‘Identity management maintenance for ex-ante and ex-post governmental policy analysis’, Proceedings of the 30th IBIMA Conference, 8-9 November 2017, Madrid, Spain, 1813-1819, ISBN 978-0-9860419-9-0
- Popescu, M.E. and Roman, M. (2018), ‘Vocational training and employability: Evaluation evidence from Romania’, Evaluation and Program Planning, 67, 38-46, https://doi.org/10.1016/j.evalprogplan.2017.11.001
- Roman, M. and Popescu, M.E. (2015), ‘The Effects of Training on Romanian Migrants’ Income: A Propensity Score Matching Approach’, Economic Computation and Economic Cybernetics Studies and Research, 43(1), 85-108.
- Sabău-Popa, D., Bradea, I., Boloș, M. and Delcea, C. (2015), ‘The information confidentiality and cyber security in medical institutions’, The Annals of the University of Oradea, 5(1), 855-860.
- Velciu, M. (2014),’Training for Changing Times’, Procedia – Social and Behavioral Sciences, 109, 220-224.
- Zamfir, A.M. and Mocanu, C. (2016), ‘Human capital, inequalities and labour market participation in Romania’, SEA-Practical Application of Science, 4(1), 135-140.