Introduction
Electronic learning is defined as the instruction or learning that is obtained via portable manipulative devices such as T.Vs (LCD, LED and Plasma), laptops, mobile phones, etc. Venkatesh et al. (2003) defined performance expectancy as the extent to which an individual believes that using a system will help him or her attain gains in job performance. In the context of this study, performance expectancy refers to the student’s belief that using e-learning will be beneficial and interesting in achieving high performance in learning. In addition, actual use or e-learning Use behaviour is defined as the physical and mental actions associated with the actual use of a system. In this study, use behaviour implies the physical and mental acts associated with the use of e-learning by students. However, the use of electronic devices such as smartphones and personal computers by students does not automatically translate into their use in education. Several models, such as the Technology Acceptance Model (TAM) and the Unified Theory of Acceptance and Use of Technology (UTAUT), have been proposed in the literature, identifying various dimensions of technology adoption especially for developed nations (Suki & Suki, 2017; Tosuntaş et al., 2015; Venkatesh et al., 2003 and Wirba Singeh, Abrizah, & Harun Abdul Karim, 2013). Since the development, integration and implementation of e-learning began in developed countries, the adoption models developed in these countries have been used as a benchmark for implementation in developing countries. Nevertheless, the determinants and barriers for adopting e-learning in different regions may or may not differ from those found in developed countries. In fact, Yakubu and Dasuki (2019) explain that the direct transfer of technological experiences from a developed country to a developing country’s context has been widely critiqued and opposed in the literature of information systems in developing countries (Avgerou, 2003; Dasuki et al., 2015 and Heeks & Bailur, 2007). Therefore, it becomes imperative to explore the determinants of e-learning adoption in the context of a developing country such as the UAE, especially from the perspective of students.
The government of the United Arab Emirates launched the largest e-learning systems in the gulf region in 2012, distributing over than 14,000 electronic devices to federal college students. Despite this massive initiative by the UAE government, Mostafa et al. (2016) report that there is a low level of acceptance towards the adoption of e-learning by students in HEIs. Notably, some of the inhibiting factors for e-learning adoption reported in the literature were language barriers, accessibility issues, culture, support, as well as personal preferences (Raman et al., 2014 and Yakubu & Dasuki, 2019). The slow-paced adoption of e-learning by students in UAE’s HEIs is worrisome, given that the infrastructural requirements for operating a robust e-learning system are in place. Thus , this calls for an investigation into the factors or determinants that enable the successful deployment and adoption of e-learning.
There is limited research on the determinants influencing students adopting e-learning in HEIS in the UAE. With the growing dependence on information systems and the increasing integration of new technologies into the learning environment, ascertaining the critical determinants related to user acceptance of technology continues to be an important issue (Tarhini et al., 2017; Tosuntaş et al., 2015 and Yakubu & Dasuki, 2019). Venkatesh et al. (2003) examined the factors that influence user acceptance of technology and identified performance expectancy, effort expectancy, social norm and facilitating conditions as the key determinants that impact the behavioural intentions to use a technology, which in turn influence their actual use. Venkatesh et al. (2003) also examined the moderating effects of age, gender, experience and perceived voluntariness of the users, in the relationship between the identified factors, behavioural intention and actual use, through the UTAUT model. Furthermore, they revealed that the UTAUT model has largely been tested in the US and suggested that the model may perform differently in other cultural settings. This, consequently, necessitates more investigation in the context of higher education in the UAE. Therefore, this study would determine the direct effects of the four determinants of e-learning adoption on the behavioural intention to use e-learning from students’ perspectives. Furthermore, the study would also examine the mediating effect of students’ behavioural intention to use e-learning in the relationship between the four determinants of e-learning adoption and the actual use of e-learning.
This study was driven by the need to address the issues surrounding the adoption of e-learning by students in UAE HEIs. The literature of e-learning is still an emerging one, and prior studies have investigated issues related to e-learning adoption in general business settings as well as in a western and developed country context, but there is a need to focus on aspects, such as higher education in a developing nation context. Hence, the overall significance of this study was to provide a clearer understanding of the determinants that influence the acceptance of e-learning technology from a student’s perspective selected from HEIs in the UAE. The findings will be an addendum to the body of literature by reporting the direct and mediating effects of the determinants of e-learning adoption and the actual use of the technology as well as providing a strong foundation that can be used to develop strategies for management and other stakeholders who are interested in a successful implementation of e-learning that students can easily adopt and use.
Literature Review
Definition of E-learning
Since the commencement of E-Learning in 1990, E-Learning has become an essential element in the educational process, transforming traditional learning environments to combine technology to construct more efficient and attractive learning experiences (Abdullah, 2011). Hence, before e-learning is broadly adopted as the name for electronic learning, several names have been given or called in the literature to other scholars, for instance, web-based training, web-based instruction, Internet-based training, distributed learning, advanced distributed learning, distance learning, online learning, mobile learning, nomadic learning, remote learning, off-site learning and web-based learning (Abdullah, 2011; Yusuf, 2013; Taha, 2014 and Mutambik, 2018). Furthermore, it is compulsory to define e-Learning to develop a clear understanding of e-Learning for institutions and educational settings, while the lack of such clear understanding is considered as an obstacle to successfully implement e-Learning (Mutambik, 2018). Many researchers and practitioners from the fields of information and communication technology, computer science, education and educational technology have contributed to defining the concept of electronic learning.
Tatweer (2014) defined e-learning as a web-based learning management system that provides different supplementary educational tools including virtual school, e- tests and self-evaluation tool, e-homework assignments tool, question bank tool and lesson planning tool for students and teachers. Zalah (2018) defined e-learning as the use of internet technologies for providing solutions that tend to improve performance and knowledge. Furthermore, Al-asmari & Khan, (2014) stated that e-Learning can be defined as the delivery of technology-supported teaching and learning, based on sound pedagogical teaching practices. “e-Learning is not a passive medium for delivery of content, but it is an interactive process between the teacher and the student, facilitated by the benefits that technology has to offer”. Hence, Mbarek and Zaddem (2013) defined e-learning as an educational and learning instruction supported by the use of the ICT, allowing learners to acquire new knowledge and skills delivered electronically without worrying about the space-time shift.
Al-Homod and Shafi (2013) stated that e-learning is an innovative approach to education delivery via electronic forms of information that enhances the learner’s knowledge, skills or other performances. In addition, e-Learning is the delivery of learning or training using electronically based approaches, mainly through the Internet, intranet, extranet, or Web. ” E-Learning involves the use of network technologies (such as Internet and business networks) for delivering, supporting and assessing formal and informal instruction” (Rao, 2011).
Unified Theory of Acceptance and Use of Technology (UTAUT)
Unified Theory of Acceptance and Use of Technology (UTAUT) concept attempts to clarify the intention of using information systems to follow-up use behavior (Vrazalic & Macgregor, 2009). The theory believes that the performance of key structures, expected value, expected workload, social inspiration and favorable environments are all through the elements of purpose and they use performance of information systems (Venkatesh et al. 2003). Further, Zhou et al. (2010) proposed that sex, age, involvement and age voluntary procedures mitigate the influence of the four key structures on use intentions and performance. UTAUT is meant to be adjusted to fit the technology being queried; therefore, a certain amount of rewording is expected. Hence, behavioral intention is defined as the person’s subjective probability that he or she will perform the behavior in question (Chang, 2013). In addition, the UTAUT model focuses on how to explain the user’s intention to use an information system and subsequent behavioral intention and identifies four key drivers of the adoption of information systems which are performance expectancy, effort expectancy, social influence and facilitating conditions. According to Lu (2014), UTAUT was tested in staged longitudinal studies and yielded strong empirical evidence of support in the social influence field. Due to this model’s functionality, the fundamental elements have been integrated into the famous expectation confirmation theory (Ayebi-Arthur, 2015). Thus, this study focuses solely on one of the four key structures of the UTAUT to examine its influence on the actual use of e-learning among higher college of technology students. The researcher used behavior intention to use e-learning as the mediation as purposed in UTAUT.
Performance Expectancy
Performance expectancy is the degree to which an individual believes that using the system will help him or her attain benefits or have high and effective performance. In other words, Performance expectancy means to what extent individuals believe their performance will improve if they adopted a system. Sair & Danish, (2018) from the work of Venkatesh et al. (2003). Thus, UTAUT model is a set or series of previous models. Five factors from previous models assisted in the formation of performance expectancy variable consisting of perceived usefulness from Technology Acceptance Models (TAM), external motivation from Motivational Model (MM), job fit from utilization model, relative advantages from innovation diffusion theory (IDT ) and an outcome from expectations social cognition theory (SCT) (Sair & Danish, 2018 and Salloum & Shaalan, 2018). Hence, several past studies uncovered that performance expectancy plays a significant role in intention to use information technology (Carter, Schaupp & McBride, 2011; Alraja, 2015 and Trybou, 2017). Venkatesh & Morris (2003) stated that by social influence, they meant the degree to which a technology user perceives that other ones are important to him or her in using a new technology. Thus, concepts of subjective norms planned behavior theory, rational action theory, technology acceptance model TAM2 and decomposed planned behavior theory. Likewise, as mentioned in performance expectancy discussion, also social factors (PC) and (IDT) were influential in the establishment of the social influence variable. According to Catherine, Geofrey, Moya & Aballo (2017), the word-of-mouth is influenced by reference people and it includes family members, friends and information technology experts, which in turn plays a main role in the acceptance or usage of information communication technology. In addition, the social influence can either be a subjective norm, social factors, or an image (Catherine et al., 2017). Ghalandari (2012) reported that perceived ease of use, personal innovativeness, perceived trust, perceived cost, social influences and perceived usefulness are key determinants influencing consumers to accept e-learning. Similarly, social influence awareness and user satisfaction are the significant predictors of the adoption of electronic learning services (Hamzat, 2018). Furthermore, Behavioural intention indicates a desire or a purpose and it is a direct determinant of the actual use of any technology (Trybou, 2017). In the UTAUT, there are two outcome variables called; behavioural intention and the actual use or behaviour. According to Arman & Hartati (2015), the intention to use an information technology can change over time and the behaviour is the actual formula of usage.
Hypotheses
H1: Performance Expectancy has a positive effect on Behavioural Intention to use e-learning.
H2: Behavioural intention mediates the relationship between performance expectancy and actual use of e-learning.
Methodology
To achieve the main objective of this study, a quantitative method was used to collect data. Based on previous studies, the questionnaire was developed taking into consideration the literature that focused on electronic learning adoption such as Rao, (2011); Ayebi-Arthur, (2015) and Homavazir, (2015). The main instrument in the questionnaire was a five-point-Likert scale, ranging from 1 strongly disagree, 2 disagree, 3 moderate, 4 agree and 5 strongly agree. The questionnaire was divided into two parts; the first is for respondents background, while the second is for covering the constructs of the study which are Performance Expectancy, Behavioural Intention to Use and Actual Use of E-learning. Before processing with the actual survey, 100 HCT’s students were selected for a pilot study to improve the questions, to test the clearness of the questions and to test the respondents’ understanding. All received comments were taken into consideration in improving the questionnaire, thus some statements were removed and corrected. Therefore, the reviewed questionnaire was distributed to a total of 490 students at Higher College of Technology in Abu Dhabi campus. Out of 490 distributed questionnaires, only 406 questionnaires were retrieved with a rate of (82.9%), this response rate
is considered a very good rate in the field of information systems.
Factor analysis and a reliability test are used to check the validity and reliability of the collected data. The results of these tests confirmed the internal consistency of constructs and measures validity. The data collected was analyzed to identify and determine the role of Behaviorual Intention to Use e-learning between Performance Expectancy and Actual Use of e-learning applications using the SPSS Version 23 and SmartPLS software. Furthermore, the next section presents the research findings obtained from the questionnaire distributed for the purpose of the research with a view to answer the main research questions of the study.
Results
The results of the study present in forms of validity and reliability of a measurement model and hypothesis testing based on the structural model to examine the mediation relationship that was proposed in the study.
Validity
Before getting the paper findings and the structural modeling, measurement model was tested in terms of its multivariate statistical analysis such as validity and reliability. It also includes loadings and cross loadings of each item used in the study.
Reliability and Validity
Table 1: Constructs Reliability and Validity
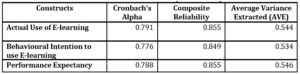
Hijazi (2017) defined reliability as the stability and consistency of the instrument used and it is one of the indicators to determine a good measure or scale. Therefore, a reliable instrument is one in which a variable or set of variables is consistent in measuring what is intended to be measured in the study (Hair and Anderson, 2010). The Cronbach alpha coefficient was used to determine the internal consistency of items in the questionnaire. Thus, all with the value of the Alpha coefficient construct more than 0.7. This means that the degree of reliability is acceptable (Bryman & Bell, 2015).
Discriminant Validity
The construct of a measure is generally celebrated from one another through a discriminant validity test. Distinct from convergent validity, discriminant validity measures items to determine that they do not unintentionally measure another variable or construct (Urbach and Ahlemann, 2010). Therefore, with regard to partial least square, two different measures are used to measure discriminant validity namely; FornellLarckers criterion and cross-loadings (Awang, 2015). The use of the FornellLarckers criterion indicates that a latent variable shares more variance with its given indicators than any other existing latent variable (Byrne, 2013).
Table 2: Discriminant Validity using Fornell and Lacker Criterion
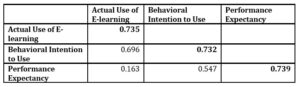
The matrix shows that for all pairwise combinations of all the latent constructs, the relationships are less than the square roots of each respective average variance extracted. Therefore, discriminant validity is achieved amongst all the constructs of the study.
Table 3: Heterotrait-Monotrait Ratio (HTMT)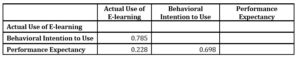
To confirm the achievement of discriminant validity in the study, the Heterotrait-Monotrait (HTMT) analysis is used to support the proposal of Fornell and Larcker, 1981). The HTMT method shows that discriminant validity is achieved when the inter-correlations between a construct of interest with all other constructs are less than 0.85 (Kline, 2015).
Table 4: Discriminant validity using cross loading criterion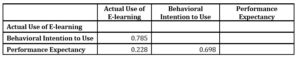
Cross-loading is derived by correlating each latent variable element score with all the other items. When an indicators’ loading is higher than that of the designated construct when compared to another related construct, this indicates that the different constructs indicators are too unique (Awang, 2015 and Dhanhani & Abdullah, 2019). According to Hair et al. (2010), the convergent validity of the measures was evaluated by the factor loadings, combined reliability and average variance extracted (AVE). The loadings of all the items exceeded the suggested value of 0.5. Therefore, based on the above results, it can be confidently concluded that the reliability and validity tests conducted on the measurement model are acceptable.
Table 5: Results of Measurement Mode
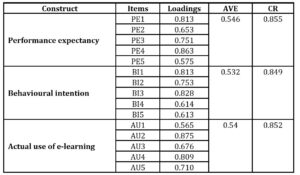
Table 5 presents the results of the measurement model showing all the three constructs namely; Performance Expectancy, Behavioural Intention to Use and Actual use of e-learningas valid constructs
Table 6: Path Coefficient and Hypothesis Testing
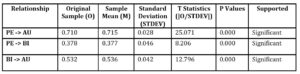
As shown in Table 6, it can be explained that Performance Expectancy has a significant effect on Actual Use of e-learning. Likewise, Performance Expectancy has a significant positive effect on Behavioural Intention to Use; also Behavioural Intonation has an effect on Actual Use of e-learning.
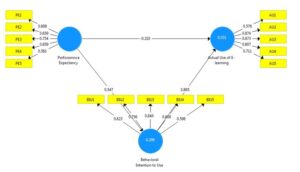
Figure 1: Measurement Model with Constructs and Indicators
Hence, the significance of such an indirect effect can be determined by the Variance Accounted for Statistic (VAF), which measures the influence of the related indirect effects on the dependent constructs, or how much of the dependent constructs is explained by the indirect effects through the mediator role. With regard to its decision rules, VAF with values that is above 80%, indicate full mediation, and values between 20% and 80% reflected partial mediation. Nevertheless, values that are below than 20% are measured as no mediation according to Hair, et al (2014). Based on the derived value of VAF, the following formula related to mediation effect is specified by Hair et al. (2014);
VAF = (p12 * p23) / (p13 + p12 * p23)
1) If 0 < VAF < 0.20, then No mediation,
2) If 0.20 < VAF < 0.80, then Partial mediation,
3) If VAF > 0.80, then Full mediation.
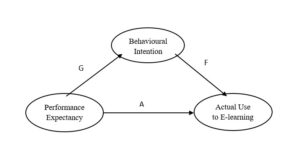
Figure 2: The Mediating role of Behavioural Intention to Use on Performance Expectancy and Actual Use of e-learning
A = 0.310
G = 0.547
F= 0.865
Indirect effects = G x F
0.547 x 0.865 = 0.473
Total effects = A + G x F = 0783
VAF (variance accounted for) indirect effects are divided by the total effect = 0.473/0.783 = 0.60. Therefore, with the VAF result of 0.60, this indicates that there is partial mediation between Performance Expectancy and Actual Use of E-learning mediated by Behavioural Intention to Use because the value derived ranges between 20% and 80%. Therefore, this implies that students at Higher College of Technology have the desire and willingness to use and adopt e-learning.
Discussion
This study investigated the influence of performance expectancy on Actual Use of e-learning using Behavioural Intention to Use as the mediation construct. The findings of the study established positive associations between performance expectancy and Actual Use of e-learning via Behavioural Intention to Use. According to Carter et al. (2011), Alraja, (2015) and Trybou (2017), performance expectancy has a significant effect on behavioural intention to use information technology. This theoretical proposal is confirmed by the outcome of this study. The present results show that performance expectancy has a positive effect on behavioral intentions to use electronic learning among the Higher College of Technology in Abu Dhabi. Thus, Performance expectancy is an analytical factor in the use of electronic learning. Likewise, this finding is in line with the submissions of Zalah (2018) in Saudi Arabia which found that behavioural intentions has positive effects on the actual use of e-learning technologies. The study also clarifies the state of e-learning in Saudi secondary schools and the role played by demographic variables, the school environment and teachers’ digital literacy. In addition, Mutambik, (2018) investigated the readiness of students and English teachers to use e-learning for English as a Foreign Language in Saudi Arabia. His results show that in developing societies, where institutions are less established and considered thinner, the introduction of e-learning in such societies will require more than the availability of financial resources and political will.
Implications
This finding implies that performance expectancy is an important ability to be developed since it can influence actual use of e-learning. Based on these findings, it is evident that electronic learning technology is a critical area that needs to be addressed by both relevant authorities and industries to increase the adoption and readiness for this system. However, as the implementation of e-learning technologies in United Arab Emirates is still moderately new, this study focused on only one of the main factors which influence the actual use of e-learning technology. Arguably, the other main elements that listed in UTAUT also merit further exploration namely; effort expectancy, social influence and facilitating conditions. It will be more interesting if further researchers can use other institutions in United Arab Emiratis to conduct such a study.
Limitations
The research may be limited in terms of the methods used in data collection, as the data was collected at one point in time. The present study focused only on one research method which is quantitative method to collect the data from respondents. The qualitative method in this study would provide a richer data in terms of how the performance expectancy and behavioural intention to use influence the actual use of e-learning applications. In term of the scope of the study, the present research was only focusing on higher college of technology’s students, which means that the findings might not be generable to other institutions in the middle east countries.
Conclusion
This research will also be of significance to the field of information systems and educational management in terms of providing insights into the direct effect of the determinants of e-learning adoption and the behavioural intention as well as the mediating effects of student’s behavioural intention to use e-learning and their actual use of e-learning. This will enable the development of an adapted model of acceptance and use of technology, which in turn can be used to better enhance the deployment and implementation of e-learning systems across HEIs in the UAE.
The study will also be beneficial to higher education stakeholders, university management and faculty members in providing the feedback needed to design efficient e-learning systems that curb the inhibiting role of language, culture, improperly designed user interfaces as well as accessibility issues. With this, effective e-learning systems can be created to allow students to tap into the enormous potentials and benefits it has to offer. It can provide university management with the necessary insights needed to formulate e-learning policies and standards for a more efficient implementation. As for faculty members, findings from the study can be used to plan for an effective integration of e-learning and the contemporary teaching and learning, taking note of the major determinants of e-learning adoption from a student’s perspective.
References
- (2011). Evaluating the E ectiveness of the E-learning Experience in Some Universities in Saudi Arabia from Male Students ’ Perceptions.
- Al-asmari, A. M., & Khan, M. S. R. (2014). E-learning in Saudi Arabia : Past , present and future.
- Alhebsi, A., Khaimah, R. Al, Pettaway, L. D., & Khaimah, R. Al. (2015). A History of Education in the United Arab Emirates and Trucial Sheikdoms, 4(1), 1–6.
- Alkaabi, S. A. R., Albion, P., & Childhood, E. (2016). Blended learning in the united arab emirates: development of an adaptability model.
- Alraja, M. N. (2015). User acceptance of information technology: A field study of an e-mail system adoption from the individual students’ perspective. Mediterranean Journal of Social Sciences, 6(6), 19-25.
- Arman, A.A., & Hartati, S. (2015). Development of user acceptance model for Electronic Medical Record system. International Conference on Information Technology Systems and Innovation, Bandung: Indonesia.
- Ati, M., & Guessoum, N. (2014). E-Learning In United Arab Emirates, (October 2010).
- Awang, Z. (2015). SEM made simple: A gentle approach to learning Structural Equation Modeling: MPWS Rich Publication.
- Ayebi-Arthur, K. (2015). Resilience, E-Learning And Change In Tertiary Education.
- Byrne, B. M. (2013). Structural equation modeling with AMOS: Basic concepts, applications, and programming: Routledge.
- Carter, L., Schaupp, L.C., McBride, M. E. (2011). The U.S. E-file initiative: An investigation of the antecedents to adoption from the individual. E-service Journal, 7(3), 2-19.
- Catherine, B. N., Geofrey, K. M., Moya, M. B., & Aballo, G. (2017). Effort Expectancy, Performance Expectancy, Social Influence and Facilitating Conditions as Predictors of Behavioural Intentions to use ATMS with Fingerprint Authentication in Ugandan Banks, 17(5).
- Dhanhani, A. Al, & Abdullah, N. H. (2019). The Effect of Transformational Leadership and Involvement on Employees ’ Job Performance in Abu Dhabi National Oil Company, 9(8), 612–621. https://doi.org/10.29322/IJSRP.9.08.2019.p9288
- Fornell, C., & Larcker, D. F. (1981). Evaluating structural equation models with unobservable variables and measurement error. Journal of marketing research, 39-50.
- Ghalandari, K. (2012). The Effect of Performance Expectancy , Effort Expectancy , Social Influence and Facilitating Conditions on Acceptance of E-Banking Services in Iran : the Moderating Role of Age and Gender, 12(6), 801–807. https://doi.org/10.5829/idosi.mejsr.2012.12.6.2536
- Hair, J., & Anderson, R. (2010). Multivariate Data Analysis 7th Pearson Prentice Hall. Prentice Hall Higher Education.
- Hair, J. F., Hult, G. T., Ringle, C., & Sarstedt, M. (2014). A Primer on Partial Least Squares Structural Equation Modeling (PLS-SEM).
- Hamzat, S. (2018). Influence of Performance Expectancy and Facilitating Conditions on use of Digital Library by Engineering Lecturers in universities in South-west , Nigeria, (February).
- Hijazi, S. (2017). The Relationship between Leadership Styles, Communication Competence and Employees ` Job Satisfaction in Private Universities of the UAE.
- Homavazir, Z. F. (2015). Impact of E- learning on student learning and employability – A study in India ” In Submitted by “ Impact of E – learning on student learning and employability – A study in India, (10).
- Iran, H. M. K. (2011). A Study on Educational Technology in Dubai Challenges and Suggested Solutions.
- Kline, R. B. (2015). Principles and practice of structural equation modeling. Guilford publications.
- Mutambik, I. (2018). Exploring the Readiness of Students and English Teachers to Use E-learning for English as a Foreign Language in Saudi Arabia.
- Rao, S. R. (2011). Global E-Learning: A Phenomenological Study.
- Sair, S. A., & Danish, R. Q. (2018). Effect of Performance Expectancy and Effort Expectancy on the Mobile Commerce Adoption Intention through Personal Innovativeness among Effect of Performance Expectancy and Effort Expectancy on the Mobile Commerce Adoption Intention through Personal Innovativeness among Pakistani Consumers, (September).
- Salloum, S. A., & Shaalan, K. (2018). Investigating students ’ acceptance of E-learning system in Higher Educational Environments in the UAE : Applying the Extended Technology Acceptance Model ( TAM ), (September).
- Suwaidi, M. Al. (2019). The Effects of Online Formative and Summative Assessment on Test Anxiety and Performance : A Study Among First-Year Undergraduate Students at A Higher Education Institution in Abu Dhabi , United Arab Emirates, (April).
- Taha, M. (2014). Investigating the Success of E-Learning in Secondary Schools: The Case of the Kingdom of Bahrain.
- Trybou, J. (2017). Performance Expectancy, Effort Expectancy and Social Influence as Factors Predicting The Acceptance of (Non-) Fluoroscopy-Guided Positioning For Radiographs, and The Relationship With Leadership, 2016–2017.
- Urbach, N., & Ahlemann, F. (2010). Structural equation modeling in information systems research using partial least squares. JITTA: Journal of Information Technology Theory and Application, 11(2), 5.
- Venkatesh, V., M. Morris, G. D. and F. D. (2003). User Acceptance of Information Technology: Toward a Unified View. MIS Quarterly, 27(3): 425-478.
- Vrazalic, L., & Macgregor, R. C. (2009). E-learning barriers in the United Arab Emirates : preliminary results from an empirical investigation E-learning barriers in the United Arab Emirates : preliminary results from, 4, 1–7.
- Yusuf, N. (2013). The Impact Of Changing Technology: The Case Of E-Learning, 6(2).
- Zahran, R., Khaimah, R. Al, & Pettaway, L. D. (2016). Educational Leadership : Challenges in United Arab Emirates, 5(1), 1–8.
- Zalah, I. (2018). Factors That Influence Saudi Secondary Teachers ’ Acceptance and Use of E-Learning Technologies.